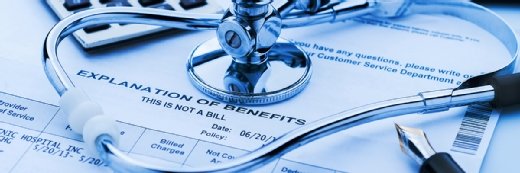
Christian Delbert - stock.adobe.
Stanford Launches Data-Driven Model Evaluating COVID-19 Interventions
The interactive, data-driven model explores the potential impact of different COVID-19 interventions.
Researchers at Stanford University have launched an interactive, data-driven model that evaluates possible outcomes of non-pharmaceutical interventions for COVID-19, including social distancing and quarantine.
For more coronavirus updates, visit our resource page, updated twice daily by Xtelligent Healthcare Media.
The team aims to help users understand the benefits of delaying the peak of the epidemic and staying below a fixed healthcare capacity. Rather than trying to map the exact dynamics of a certain location, the model shows possible trajectories under different hypothetical scenarios.
“We wanted to start a larger conversation about how our long-term response might look,” said Erin Mordecai, Stanford biologist. “We’re concerned about the potential for the disease to rapidly spread once we lift control measures.”
The modeling framework allows for different types, intensities, and durations of interventions to be implemented, and shows how these different interventions impact the number of COVID-19 cases and fatalities through time.
Researchers considered three possible interventions that can be implemented at different times in the model simulation, including social distancing for a set duration; social distancing triggered by the number of hospitalized individuals crossing a threshold; and isolation of symptomatic individuals.
“In the future, we are considering additional interventions and scenarios including contact tracing with efficacy dependent on the testing capacity, fatality and hospitalization rates dependent on the age structure of a population, and fatality rates further dependent on hospital capacities,” researchers said.
The team also noted that the effects of these interventions will heavily depend on transmission rate, which varies from region to region. Additionally, researchers pointed out that for short-term interventions to be effective, much higher levels of social distancing will likely be required. People shouldn’t expect to stop these measures once the number of COVID-19 cases starts to decline, either.
“If we lift controls too quickly, we could see a resurgence, where cases pick back up quickly. In fact, if we completely stop practicing social distancing at almost any point in this model, we risk an epidemic that overwhelms hospital capacity,” the group stated.
“We will probably have to stay careful for a long time after it seems like COVID-19 is gone in order to keep it under control, or until a vaccine is developed (which could take twelve to eighteen months). That doesn't mean we will necessarily need to stay in our houses the whole time, though.”
Keeping transmission relatively low will likely depend on what the team referred to as the “lightswitch” method. This means that when regions are on, they go back to social distancing and cases start to decrease. When regions turn it off, people can take a break from social distancing and make smaller adjustments instead. The number of cases may start to increase a bit, researchers said, but won’t get out of control before places go back to social distancing.
As the pandemic has progressed, many organizations have leveraged their big data resources to track and predict the spread of COVID-19.
Researchers at UTHealth recently developed an artificial intelligence tool that showed immediate stringent interventions are needed to curb the spread of coronavirus in the Greater Houston area.
“Although there are a lot of numbers and a lot of details, we saw two consistent patterns: earlier intervention was better, and more stringent intervention was better than less stringent,” said Eric Boerwinkle, PhD, dean and M. David Low Chair in Public Health at UTHealth School of Public Health. “It is also heartening to see that Texas generally fares better than many states in the top tier of case numbers.”
With the launch of this data-driven model, Stanford researchers hope to shed light on best practices for reducing and mitigating the impact of COVID-19 in the US.
“We know this is an incredibly stressful and scary time for people around the world. There's a lot of uncertainty, but scientists, medical professionals, and leaders across industries are working together to help us find solutions,” researchers concluded.
“If we make decisions based on the best models we have, we can get through this crisis while protecting the most vulnerable and minimizing the impact on our lives and the economy.”