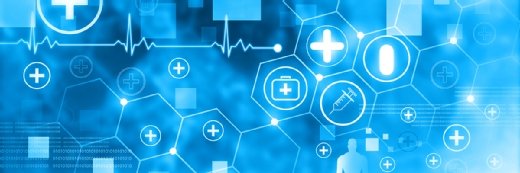
Rasi Bhadramani/istock via Getty
Machine Learning Tool Uses Real-Time Data to Monitor Flu Trends
The portable device uses machine learning to detect coughing sounds and crowd size in real time, directly monitoring flu-like illnesses and flu trends.
Researchers at the University of Massachusetts Amherst (UMass Amherst) have developed a portable surveillance tool that leverages machine learning and real-time data to monitor flu-like illnesses and flu patterns.
The device, called FluSense, can detect coughing sounds and crowd size in real time, and could add to the collection of tools used to forecast seasonal flu and other viral outbreaks. The team recently published the results of their research in the journal Proceedings of the Association for Computing Machinery on Interactive, Mobile, Wearable and Ubiquitous Technologies.
“This may allow us to predict flu trends in a much more accurate manner,” said Tauhidur Rahman, assistant professor of computer and information sciences and co-author of the study.
FluSense is run on an edge-computing platform, and processes information from a low-cost microphone and thermal imaging data. The platform stores no personally identifiable information like speech data or distinguishing images.
The team first developed a lab-based cough model, then trained a deep neural network classifier to draw bounding boxes on thermal images representing people and then to count them. Researchers then placed FluSense devices in four healthcare waiting rooms at UMass’s University Health Services clinic.
The FluSense platform collected and analyzed more than 350,000 thermal images and 21 million non-speech audio samples from public waiting areas between December 2018 and July 2019.
The results showed that the platform was able to accurately predict daily illness rates at the university clinic. Multiple and complementary sets of FluSense signals strongly correlated with lab-based testing for flu-like illnesses and influenza itself.
The researchers believe the tool could add valuable information to current influenza prediction efforts, including the FluSight Network, a group that uses predictive analytics models to forecast trends in influenza outbreaks with greater accuracy than historical baseline models.
“Our main goal was to build predictive models at the population level, not the individual level,” Rahman said.
“I thought if we could capture coughing or sneezing sounds from public spaces where a lot of people naturally congregate, we could utilize this information as a new source of data for predicting epidemiologic trends.”
FluSense creators also noted that the tool could help forecast other respiratory outbreaks, such as the COVID-19 pandemic or SARS.
With the rapid spread of COVID-19 across the US, policymakers have urged experts in AI and data analytics to develop tools that will help track and control the virus. The White House Office of Science and Technology Policy recently issued a call to action for researchers to build AI tools that can be applied to a new COVID-19 dataset.
“Decisive action from America’s science and technology enterprise is critical to prevent, detect, treat, and develop solutions to COVID-19. The White House will continue to be a strong partner in this all hands-on-deck approach,” said Michael Kratsios, US Chief Technology Officer, the White House.
“We thank each institution for voluntarily lending its expertise and innovation to this collaborative effort, and call on the United States research community to put artificial intelligence technologies to work in answering key scientific questions about the novel coronavirus.”
Innovative tools like FluSense, which combines AI and edge computing to enable real-time data analytics, could help accelerate outbreak tracking and understanding. Models like these could directly inform the public during a flu epidemic, and could help determine the timing necessary for flu vaccine campaigns, potential travel restrictions, or the allocation of medical supplies.
“We are trying to bring machine-learning systems to the edge,” said Forsad Al Hossain, PhD student and lead author of the study. “All of the processing happens right here. These systems are becoming cheaper and more powerful.”
The researchers plan to further develop and refine FluSense by testing it in other public areas and geographic locations.
“We have the initial validation that the coughing indeed has a correlation with influenza-related illness,” said Andrew Lover, a vector-borne disease expert and assistant professor in the School of Public Health and Health Sciences “Now we want to validate it beyond this specific hospital setting and show that we can generalize across locations.”