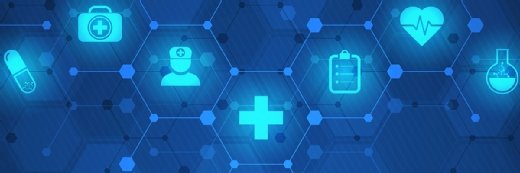
bestbrk/istock via Getty Images
Machine Learning Approach Could Accelerate COVID-19 Drug Discovery
By combining machine learning and quantum physics, researchers could speed the process of drug discovery for COVID-19.
Researchers from Penn State College of Engineering are aiming to speed COVID-19 drug discovery with quantum machine learning, a field that combines machine learning and quantum physics.
For more coronavirus updates, visit our resource page, updated twice daily by Xtelligent Healthcare Media.
The researchers noted that the existing drug-discovery pipeline can take five to ten years from initial idea to market approval, and could cost billions of dollars. The team believes that quantum machine learning approach could be faster and more economical than current methods used for drug discovery.
“Discovering any new drug that can cure a disease is like finding a needle in a haystack,” said Swaroop Ghosh, the Joseph R. and Janice M. Monkowski Career Development Assistant Professor of Electrical Engineering and Computer Science and Engineering.
“High-performance computing such as supercomputers and artificial intelligence can help accelerate this process by screening billions of chemical compounds quickly to find relevant drug candidates.”
Using quantum machine learning, the research team will seek to uncover new ways to discover and develop disease treatments.
“This approach works when enough chemical compounds are available in the pipeline, but unfortunately this is not true for COVID-19. This project will explore quantum machine learning to unlock new capabilities in drug discovery by generating complex compounds quickly,” said Ghosh.
Ghosh and his team have previously worked on developing a toolset for solving particular types of problems, known as combinational optimization problems, using quantum computing. Drug discovery falls into the same type of problem as those they previously worked on, which made it possible for the researchers to transition to looking for a COVID-19 treatment while using the same toolset they had already developed.
“Artificial intelligence for drug discovery is a very new area. The biggest challenge is finding an unknown solution to the problem by using technologies that are still evolving — that is, quantum computing and quantum machine learning,” said Ghosh.
“We are excited about the prospects of quantum computing in addressing a current critical issue and contributing our bit in resolving this grave challenge.”
Researchers across the healthcare continuum have started to leverage AI and other big data analytics tools to quickly find treatments for COVID-19.
In April 2020, the Harvard T.H. Chan School of Public Health and the Human Vaccines Project launched the Human Immunomics Initiative, which uses artificial intelligence models to accelerate vaccine development. Using these models, researchers can virtually test potential vaccines and predict which therapies might work best across populations.
“The way we fight disease is broken—we launch into disease-specific battles without understanding the rules that affect our chances of success,” said Michelle A. Williams, Dean of Harvard T.H. Chan School of Public Health.
“The Human Vaccines Project set out to change that by decoding the human immunome, and we are already seeing initial findings from their tremendous work. We are excited to bring the intellectual and technological resources of Harvard Chan School, and our colleagues across Harvard, to further those efforts through HII.”
Two graduates from Columbia University also recently launched a startup that creates machine learning algorithms capable of computationally generating, screening, and optimizing hundreds of millions of therapeutic antibodies.
This method could significantly speed the amount of time it takes to discover new treatments and bring them to market, the researchers said.
“What our algorithms do is reduce the likelihood of drug-discovery failure in the lab,” said Andrew Satz, a graduate of the Data Science Institute at Columbia University. “We fail in the computer as much as possible to reduce the possibility of downstream failure in the laboratory. And that shaves a significant amount of time from laborious and time-consuming work.”