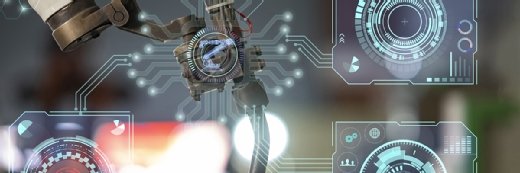
Getty Images/
Top 5 Use Cases for Artificial Intelligence in Medical Imaging
Artificial intelligence can support radiologists and pathologists as they use medical imaging to diagnose a wide variety of conditions.
Artificial intelligence and machine learning have captivate the healthcare industry as these innovative analytics strategies become more accurate and applicable to a variety of tasks.
AI is increasingly helping to uncover hidden insights into clinical decision-making, connect patients with resources for self-management, and extract meaning from previously inaccessible, unstructured data assets.
Medical imaging data is one of the richest sources of information about patients, and often one of the most complex.
With megapixel upon megapixel of data packed into the results from X-rays, CAT scans, MRIs, and other testing modalities, combing through extremely high-resolution images can be challenging even for the most experienced clinical professional.
Artificial intelligence has already proven that it may be a valuable ally for radiologists and pathologists looking to accelerate their productivity and potentially improve their accuracy.
Multiple studies have indicated that AI tools can perform just as well, if not better, than human clinicians at identifying features in images quickly and precisely.
Far from being a matter of concern for the American College of Radiology, the advent of AI as a companion for diagnosticians is a positive development, leaders of the society said.
In order to foster standardized, safe, and effective AI for clinical decision support and diagnostics, the American College of Radiology Data Science Institute (ACR DSI) has released a number of high-value use cases for artificial intelligence in medical imaging, which will be continuously updated as new opportunities present themselves.
“The ACR DSI use cases present a pathway to help AI developers solve health care problems in a comprehensive way that turns concepts for AI solutions into safe and effective tools to help radiologists provide better care for our patients,” said Bibb Allen Jr., MD, FACR, ACR, Chief Medical Officer at DSI.
As part of the ACR DSI Technology Oriented Use Cases in Health Care-AI (TOUCH-AI) Framework, the use cases offer real-world scenarios in which AI tools can supplement and enhance the process of using medical images to deliver high-quality patient care across a wide variety of diseases and organ groups.
What are the top five use cases for artificial intelligence in the imaging world, and how can AI tools alter workflows to improve the detection and diagnosis of potentially fatal conditions?
Identifying cardiovascular abnormalities
Measuring the various structures of the heart can reveal an individual’s risk for cardiovascular diseases or identify problems that may need to be addressed through surgery or pharmacological management.
Automating the detection of abnormalities in commonly-ordered imaging tests, such as chest x-rays, could lead to quicker decision-making and fewer diagnostic errors.
For example, when a patient enters the emergency department with a complaint such as shortness of breath, “the chest radiograph is often the first imaging study that is available,” ACR DSI says.
“It can be used as a quick initial screening tool for cardiomegaly, which in and of itself can be used as a marker for heart disease. A quick visual assessment by a radiologist is sometimes inaccurate.”
Using artificial intelligence to identify left atrial enlargement from chest x-rays could rule out other cardiac or pulmonary problems and help providers target appropriate treatments for patients.
Similar AI tools could be used to automate other measurement tasks, such as aortic valve analysis, carina angle measurement, and pulmonary artery diameter.
Applying AI to imaging data may also help to identify thickening of certain muscle structures, such as the left ventricle wall, or monitor changes in blood flow through the heart and associated arteries.
“Automated pulmonary artery flow quantification would save the interpreting physician time via elimination of manual measurements, prevent detection errors, and provide structured quantitative data, which could be used in later studies or risk stratification schemes,” ACR DSI suggests.
Algorithms could automatically populate reports, saving time for human clinicians, and identify measurements or values that qualify as abnormal.
Detecting fractures and other musculoskeletal injuries
Fractures and musculoskeletal injuries can contribute to long-term, chronic pain if not treated quickly and correctly.
Injuries such as hip fractures in elderly patients are also tied to poor overall outcomes due to reductions in mobility and associated hospitalizations.
Using artificial intelligence to identify hard-to-see fractures, dislocations, or soft tissue injuries could allow surgeons and specialists to be more confident in their treatment choices.
After trauma, fractures are often considered secondary in importance, says ACR DSI, at least compared to internal bleeding or organ injury. When human diagnosticians looking at trauma-related imaging focus first on their immediate clinical concerns, fractures can sometimes be overlooked.
In one example, a patient presenting to the ED with head and neck trauma could be assessed for odontoid fracture – a type of fracture in the cervical spine – by using an AI radiology tool.
The fracture type is often difficult to detect on standard images, but AI tools may be more likely to see subtle variations in the image that could indicate an instability that requires surgery.
Allowing unbiased algorithms to review images in trauma patients may help to ensure that all injuries are accounted for and receive the care required to secure a positive outcome.
Providers may also find that AI provides a useful safety net when conducting routine follow-ups for common hip surgeries, such as hip joint replacements.
“There are roughly 400,000 total hip arthroplasties (THAs) performed annually,” ACR DSI says. “Every patient has annual follow-up exams, which can add up to about 100 exams per day for a musculoskeletal radiologist who works with arthroplasty surgeons.”
If a joint replacement device becomes loosened or the tissue around the device reacts poorly, the patient could require an expensive and invasive revision.
Unfortunately, identifying problems around the site can be challenging.
“Findings are not readily apparent on x-ray and require comparison with multiple prior exams to see progression of abnormality over time,” says ACR DSI. “Delay in diagnosis could delay treatment for years.”
“AI meeting this use case would help to reduce the false negative rate, patient risk, and medical legal risk for the radiologists. High-risk patients could be screened for elevated serum cobalt levels and sent to MRI for further evaluation.”
Aiding in the diagnosis neurological diseases
Degenerative neurological diseases, such as amyotrophic lateral sclerosis (ALS), can be a devastating diagnosis for patients. While there is currently no cure for ALS and many similar neurological conditions, accurate diagnoses could help individuals understand their likely outcomes and plan for long-term care or end-of-life wishes.
Identifying ALS – and distinguishing between ALS and primary lateral sclerosis (PLS) – relies on imaging studies, says the College. Radiologists must decide if lesions are relevant or simply mimicking the structures of one of the diseases, and false positives are relatively common.
Recent research into improving the speed and accuracy of diagnoses has focused on identifying new biomarkers.
“Currently, manual segmentation and quantitative susceptibility mapping (QSM) assessments of the motor cortex are necessary, difficult, and time consuming,” ACR DSI says. “Automating this procedure with machine learning would facilitate research and assist in the development of a promising imaging biomarker.”
Algorithms may be able to streamline this process by flagging images that indicate suspect results and offering risk ratios that the images contain evidence of ALS or PLS. Algorithms may also be able to automatically populate reports, reducing workflow burdens on providers.
Flagging thoracic complications and conditions
Pneumonia and pneumothorax are two conditions that require quick reactions from providers. Both may also be prime targets for artificial intelligence algorithms.
Pneumonia, either acquired in the community or after a medical procedure, can be life threatening if left untreated. Radiology images are often used to diagnose pneumonia and distinguish the condition from other lung conditions, such as bronchitis.
Yet radiologists may not always be available to read images – and even if radiologists are present, they may have difficulty identifying pneumonia if the patient has pre-existing lung conditions, such as malignancies or cystic fibrosis.
In addition, “subtle pneumonias, such as those projecting below the dome of the diaphragms on front chest radiographs, can easily be overlooked and lead to unnecessary CT scans, which AI could help reduce,” ACR DSI says.
An AI algorithm could assess x-rays and other images for evidence of opacities that indicate pneumonia, then alert providers to the potential diagnoses to allow for speedier treatment.
AI can similarly help to identify high-risk patients when pneumothorax is suspected, especially when radiologists are not present.
Pneumothorax, or the introduction of air pockets between the lung and chest wall, can be the result of trauma or invasive interventions. While the condition is treatable, it can be serious is left undetected.
“In clinical settings where a radiologist is not readily available, detection [of pneumothorax] would be of value to non-radiologists,” ACR DSI points out.
Artificial intelligence may be able to help prioritize the type and severity of pneumothoraces, which may change the urgency of treatment.
AI may also be able to help providers monitor patients over time.
“One possible extension to this use case is to track changes in size of previously detected pneumothoraces and those under treatment,” ACR DSI suggests.
Screening for common cancers
Medical imaging is often used in routine, preventive screenings for cancers, such as breast cancer and colon cancer.
In breast cancer, microcalcification in tissue can often be difficult to conclusively identify as either malignant or benign. False positives could lead to unnecessary invasive testing or treatment, while missed malignancies could result in delayed diagnoses and worse outcomes.
“There is variability in radiologist interpretation…of microcalcifications at the time of diagnostic imaging,” ACR DSI says.
“AI can help improve accuracy and use quantitative imaging features to more accurately categorize microcalcifications by level of suspicion for ductal carcinoma in situ (DCIS), potentially decreasing the rate of unnecessary benign biopsies.”
Providing risk scores for areas of concern could allow providers and patients to make more informed decisions about how to proceed with testing or treatment.
Similarly, patients undergoing screenings for colorectal cancer may have more productive conversations with their providers if polyps are found during routine checks. Polyps are precursors to cancer.
“CT colonography (CTC) provides a minimally invasive structural exam of the colon and rectum to detect clinically significant polyps,” ACR DSI explains. “However, less experienced radiologists can miss polyps and take excessive time to complete the exam. AI could help improve accuracy and efficiency of polyp detection at CTC, reduce false positives, and reduce medical legal risk for radiologists.”
For patients with established cancers, AI could support the detection of malignancies that have spread. Extranodal extension (ECE) of cancers is associated with poor prognosis, and is often only discovered at the time of a surgery.
“A performant algorithm could potentially identify ECE for diagnoses that do not usually proceed to surgery, potentially enabling better treatment stratification in this population. Automated ECE classification and identification could also enable improved radiotherapy targeting of nodal basins, as well as treatment optimization for post-operative imaging-detected nodal disease,” says ACR DIS.
AI could be useful for head and neck cancers, prostate cancer, colorectal cancers, and cervical cancer, the society says.
“Although not proven, this algorithm or a semi-automated approach could improve cancer outcomes and decrease morbidity,” ACR DSI added.
While more study will be required to test the utility of AI for these and other use cases, ACR DIS appears confident that medical imaging is ready for artificial intelligence. Supplementing diagnostics and decision-making with AI could offer providers and patients life-changing insights into a variety of diseases, injuries, and conditions that may be difficult to identify with the human eye alone.