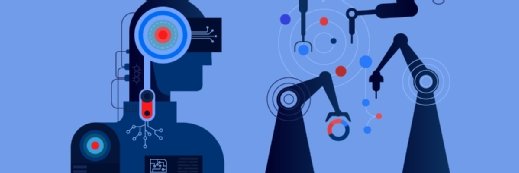
Getty Images
Artificial Intelligence Detects Epileptic Seizures in Real Time
An artificial intelligence system was able to efficiently and accurately identify epileptic seizures in real time.
An artificial intelligence algorithm can analyze electroencephalograph (EEG) electrodes to detect a seizure and accurately pinpoint its location, according to a study published in Scientific Reports.
The researchers stated that epilepsy is one of the most common central nervous system disorders, with nearly four percent of people across different ages diagnosed with epilepsy during their lifetimes.
The current understanding of most seizures is that they occur when normal brain activity is interrupted by a strong, sudden hyper-synchronized firing of a cluster of neurons. During a seizure, if a person is hooked up to an EEG – a device that measures electrical output – the abnormal brain activity is presented as amplified spike-and-wave discharges.
However, when temporal EEG signals are used, it can be difficult to accurately detect a seizure. Researchers developed a network inference technique that would facilitate detection of a seizure and pinpoint its location with improved accuracy.
During an EEG session, a person has electrodes attached to different spots on her head, and each electrode records electrical activity around that spot.
“We treated EEG electrodes as nodes of a network. Using the recordings (time-series data) from each node, we developed a data-driven approach to infer time-varying connections in the network or relationships between nodes,” said Walter Bomela, a postdoctoral fellow in the Preston M. Green Department of Electrical & Systems Engineering at the University of Texas at Arlington. “We want to infer how a brain region is interacting with others.”
These relationships form a network. Once researchers had a network, they could measure its parameters holistically. For example, instead of measuring the strength of a single signal, the team could evaluate the overall network for strength.
One parameter, the Fiedler eigenvalue, starts to increase when a seizure occurs. In network theory, the Fiedler eigenvalue is also related to a network’s synchronicity – the bigger the value the more the network is synchronous.
“This agrees with the theory that during seizure, the brain activity is synchronized,” Bomela said.
A bias toward synchronization also helps to reduce artifact and background noise, researchers noted. For instance, if a person scratches their arm, the associated brain activity will be captured on some EEG channels or electrodes. However, it won’t be synchronized with seizure activity. This structure inherently eliminates the importance of unrelated signals, so that only brain activities that are in sync will significantly increase the Fiedler eigenvalue.
“Our technique allows us to get raw data, process it and extract a feature that’s more informative for the machine learning model to use,” said Bomela. “The major advantage of our approach is to fuse signals from 23 electrodes to one parameter that can be efficiently processed with fewer computing resources.”
Currently, the system works for an individual patient. The next step is to integrate machine learning to generalize the technique for identifying different types of seizures across patients. Researchers are seeking to take advantage of various parameters characterizing the network and use them as features to train the machine learning algorithm.
“The network is like a face,” said Bomela. “You can extract different parameters from an individual’s network — such as the clustering coefficient or closeness centrality — to help machine learning differentiate between different seizures.”
In network theory, similarities in specific parameters are associated with specific networks. In this case, those networks will correspond to different types of seizures.
The team’s overall aim is to one day design a device for people with epilepsy that is analogous to an insulin pump. As the neurons begin to synchronize, the device will provide medication or electrical interference to stop the seizure. However, in order for this to happen, researchers need a better understanding of the neural network.
“While the ultimate goal is to refine the technique for clinical use, right now we are focused on developing methods to identify seizures as drastic changes in brain activity,” said Jr-Shin Li, professor in the Preston M. Green Department of Electrical & Systems Engineering. “These changes are captured by treating the brain as a network in our current method.”