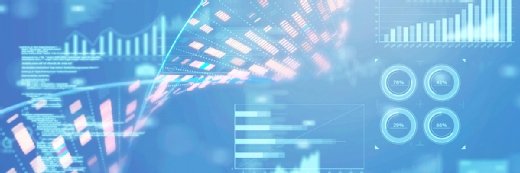
Yuichiro Chino/Moment via Getty
Machine Learning Uses EHR Data to Predict Alzheimer’s Risk
Machine learning algorithms analyzed EHR data and accurately predicted the onset of dementia within one to three years of diagnosis.
Using structured and unstructured EHR data, machine learning algorithms could accurately identify patients at risk of developing Alzheimer’s disease and related dementias.
At least 50 percent of older primary care patients living with Alzheimer’s disease and related dementias never get diagnosis, researchers stated, and many more live with symptoms for two to five years before being diagnosed. Current tests that screen for dementia risk are invasive, time-consuming, and invasive.
The research team, which included scientists from Regenstrief Institute, Georgia State, Albert Einstein College of Medicine, and Solid Research Group, used two different machine learning approaches to improve dementia and Alzheimer’s diagnosis: A natural language processing algorithm and a random forest model.
To train the algorithms, researchers gathered data from the Indiana Network for Patient Care. The models analyzed information in structured fields, such as prescriptions and diagnoses, as well as unstructured fields like medical notes. In predicting dementia development, researchers found that the medical notes were the most valuable resource for helping to identify high-risk patients.
“The great thing about this method is that it’s passive, and it provides similar accuracy to the more intrusive tests that are currently used,” said lead researcher Malaz Boustani, MD, MPH, a research scientist at Regenstrief Institute and a professor at Indiana University School of Medicine.
“This is a low cost, scalable solution that can provide substantial benefit to patients and their families by helping them prepare for the possibility of life with dementia and enabling them to take action.”
These methods can provide significant cost savings for patients and health systems, replacing the need for expensive tests and allowing clinicians to screen entire populations to identify those at risk. By delaying the onset of symptoms, healthcare organizations, patients, and their families will also save a significant amount on treatment.
“This research is exciting because it potentially provides significant benefit to patients and their families,” said Patrick Monahan, PhD, study author from IU School of Medicine and a Regenstrief affiliate scientist. “Clinicians can provide education on behavior and habits to help patients cope with their symptoms and live a better quality of life.”
Future work will involve deploying these machine learning algorithms in real-life clinics to see if they help identify more true cases of dementia and learn how they impact a patient’s willingness to follow up on the results.
“The early risk identification allows an opportunity for doctors and families to put a care plan in place. I know from experience what a burden it can be to deal with a dementia diagnosis. The window provided by this test is so important to help improve the quality of life for both patients and their families,” said Zina Ben Miled, PhD, MS, a study author from the Purdue School of Engineering and Technology at IUPUI and a Regenstrief affiliate scientist.
Researchers are increasingly using artificial intelligence models to improve Alzheimer’s treatment and diagnosis. Recently, a team from the Massachusetts Institute of Technology (MIT) developed artificial intelligence tools to determine whether patients at high risk of Alzheimer’s disease will experience cognitive decline.
The models could help select candidate drugs and participant cohorts for clinical trials, and allow patients and their families prepare for cognitive decline.
“Accurate prediction of cognitive decline from six to 24 months is critical to designing clinical trials,” said Oggi Rudovic, a Media Lab researcher. “Being able to accurately predict future cognitive changes can reduce the number of visits the participant has to make, which can be expensive and time-consuming. Apart from helping develop a useful drug, the goal is to help reduce the costs of clinical trials to make them more affordable and done on larger scales.”