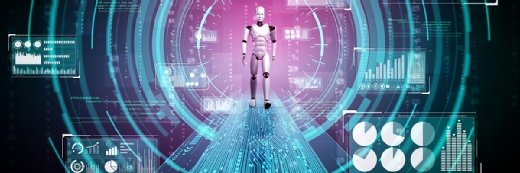
Getty Images
Machine Learning Models Estimate Seasonal Impact of COVID-19
Researchers will apply machine learning algorithms to environmental data to determine whether COVID-19 surges and wanes in different seasons.
Using machine learning, scientists may be able to assess the role climate and environmental variables play in the transmission of COVID-19.
For more coronavirus updates, visit our resource page, updated twice daily by Xtelligent Healthcare Media.
A team from Lawrence Berkeley National Laboratory is applying machine learning methods to health and environmental datasets to determine whether the virus fades in warmer months and surges once it gets colder – similar to the flu.
“Environmental variables, such as temperature, humidity, and UV exposure, can have an effect on the virus directly, in terms of its viability. They can also affect the transmission of the virus and the formation of aerosols,” said Berkeley Lab scientist Eoin Brodie, the project lead.
“We will use state-of-the-art machine-learning methods to separate the contributions of social factors from the environmental factors to attempt to identify those environmental variables to which disease dynamics are most sensitive.”
Researchers will leverage county-level data, including the severity, distribution, and duration of the COVID-19 outbreak, and what public health interventions were issued when. The team will also analyze demographics, climate and weather factors, and population mobility dynamics.
The initial goal of the project is to predict how environmental factors can impact the transmission of COVID-19 in each US county. This will require the research team to integrate data across scales in order to make predictions at the local level.
“Downscaling of climate information is something that we routinely do to understand how climate impacts ecosystem processes,” Brodie said. “It involves the same types of variables – temperature, humidity, solar radiation.”
The cross-disciplinary team includes scientists with expertise in climate modeling, data analytics, machine learning, and geospatial analytics. A principal aim of the study is to understand how climate and weather interact with societal factors.
“We don’t necessarily expect climate to be a massive or dominant effect in and of itself. It’s not going to trump which city shut down when. But there may be some really important interactions between the variables,” said Ben Brown, a computational biologist in Berkeley Lab’s Biosciences Area, who is leading the machine-learning analysis.
“Looking at New York and California for example, even accounting for the differences between the timing of state-instituted interventions, the death rate in New York may be four times higher than in California – though additional testing on random samples of the population is needed to know for sure. Understanding the environmental interactions may help explain why these patterns appear to be emerging. This is a quintessential problem for machine learning and AI.”
Temperature, humidity, and UV index have all been statistically associated with rates of COVID-19 transmission, the team noted, although contact rates are still the dominant impact on the spread of the virus. In the southern hemisphere, where it is currently fall, the spread has been slower than in the northern hemisphere.
“There’s potentially other factors associated with that,” Brodie said. “The question is, when the southern hemisphere moves into winter, will there be an increase in transmission rate, or will fall and winter 2020 lead to a resurgence across the US in the absence of interventions?”
Researchers also pointed out that in India, COVID-19 does not seem to be as strong.
“There are cities where it behaves as if it’s the most infectious disease in recorded history. Then there are cities where it behaves more like influenza,” Brown said. “It is really critical to understand why we see those massive differences.”
The team believes that there is now enough data available to understand how environmental factors influence the spread of COVID-19. Researchers expect to have the first phase of their analysis available by late summer or early fall. The next phase will involve making projections under different scenarios, which could help inform public health decisions.
“We would use models to project forward, with different weather scenarios, different health intervention scenarios – such as continued social distancing or whether there are vaccines or some level of herd immunity – in different parts of the country,” said Brodie.
“For example, we hope to be able to say, if you have kids going back to school under this type of environment, the climate and weather in this zone will influence the potential transmission by this amount. That will be a longer-term task for us to accomplish.”