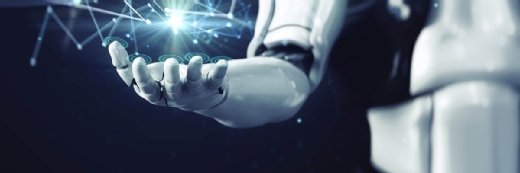
Getty Images
Testing System Leverages Machine Learning to Predict Sepsis Outcomes
A new testing approach uses machine learning to accurately identify which sepsis patients will recover quickly, recover later, or die from the condition.
Using machine learning algorithms, an extremely sensitive method can analyze biomarkers to predict sepsis outcomes with nearly 100 percent accuracy, according to a study published in Nature Communications.
More than 1.7 million Americans develop sepsis each year, and more than 270,000 die from it, researchers noted. Although there have been advancements in early recognition and supportive care, there are still few strategies for effectively treating sepsis.
“Despite years of research, diagnosis and prognostication of sepsis and septic shock predominantly rely on clinical criteria. Numerous biomarkers have been studied, but in isolation, none of them have the accuracy necessary to be used in clinical practice,” researchers said.
“In order to identify patients most likely to benefit from targeted interventions, new tools are needed that allow accurate characterization of the diverse pathogen and host factors that shape outcomes for patients with sepsis and septic shock.”
Sepsis is often caused by microbial infections and is usually initially treated with antibiotics. Any delay in the administration of correct antibiotics can significantly increase the chances of the patient dying, the team stated.
“Every hour delay in administration of appropriate antibiotics results in a 4% increase in odds of death,” researchers said.
“Strategies to improve sepsis and septic shock outcomes must include the rapid identification of infectious pathogens and their antibiotic-resistance patterns, which typically depends on culturing of relevant biological samples.”
Even if clinicians can treat the infection directly, the condition can cause the body’s immune response to become exaggerated. This can result in the immune system releasing too many immune system proteins called cytokines, which can ultimately overwhelm the body and kill the patient.
Researchers from the University of Chicago developed a new, extremely sensitive method that can quantify bacteria, an antibiotic resistant gene, and immune molecule levels within sepsis patients more rapidly than current approaches.
The team developed a digital polymerase chain reaction (PCR) test that uses digital proximity litigation assays to quantify the levels of particular proteins and genes in the blood. Specifically, the method uses a blood sample to test for gram-negative (GN) and gram-positive (GP) bacterial DNA, which is abundant in many septic patients.
The approach also tests for levels of the IL-6 and TNF proteins, the cytokines that the immune system releases to attack pathogens. Additionally, it tests for the blaTEM gene, which signifies antibiotic resistance.
To develop the test, the group took samples once a day for two days from 32 patients and tested their bacterial and protein levels. The results showed that the bacterial levels of the patients who lived decreased as time went on.
However, the findings also showed that in almost every patient that died, IL-6 levels increased throughout their time at the hospital. Even patients who had low bacterial levels to begin with still died if their IL-6 levels increased, demonstrating that the immune system potentially overshot and attacked their own body.
While IL-6 has been a major biomarker in sepsis before, past research didn’t show that it was the change in the levels – not the levels themselves – that predicted this outcome. The team also found several patients with the gene that indicates antibiotic resistance, which is helpful for physicians treating them.
“Sepsis manifests itself differently in each person, therefore having a test like this to shed light on that variation could one day be used by providers to identify which patients may respond better to certain treatments or interventions,” said Krysta Wolfe, a pulmonologist and assistant professor of medicine at UChicago, and co-author of the research.
Using machine learning algorithms, researchers could ultimately use these biomarkers to predict who could recover early, recover late, or die with nearly 100 percent accuracy.
The team is working to develop a machine that can quickly test samples on site at ICUs. Researchers are proceeding with a clinical trial and hope to extend the test to include more groups of bacteria beyond just the GN and GP levels. This will help providers better understand which antibiotics are needed to reduce antibiotic resistance.
The test could also be extended to other infections where cytokines can overtake the body, including viral infections like COVID-19.
“Our findings provide a new approach to the diagnosis of sepsis with the potential to identify the causal pathogen early,” said Gokhan Mutlu, professor of medicine and chief of pulmonary and critical care medicine at UChicago and co-author of the research.
“This will allow us to use the appropriate antibiotics earlier before the culture results are available and minimize the use of antibiotics that are needed to treat the infection. By combining the pathogen-related and host response data, we are able to predict outcomes in patients with sepsis.”