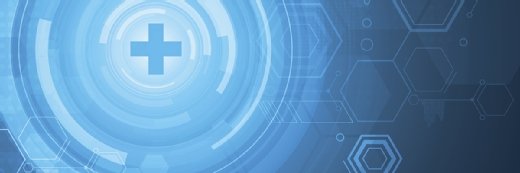
traffic_analyzer/DigitalVision V
Predictive Analytics Models Forecast Prevalence of Flu Strains
The predictive analytics models examine virus’ genetic codes to understand how the flu will evolve, potentially leading to more protective vaccines.
Predictive analytics models that evaluate genetic and experimental data could more accurately forecast which flu strains will be most prevalent during the next winter, according to a study published in eLife.
The models have the potential to make flu vaccines more accurate and protective, resulting in fewer illnesses and deaths.
Seasonal flu infects between five and 15 percent of the world’s population each year, causing between a quarter of a million and half a million deaths, researchers stated. While vaccination is the best protection against seasonal flu, the flu virus changes its molecular appearance each year, meaning the virus is able to get past the immune defenses learned from the year before.
Every year, the vaccine needs updating. But it takes almost a year to design a new flu vaccine, researchers have to be able to predict what flu viruses will look like in the future. The current prediction approach relies on experiments that assess the molecular appearance of flu viruses, particularly at a key molecule that coats the virus called haemagglutinin.
Examining the virus’s genetic code presents a faster, more streamlined approach, the team said. Predictive analytics models can determine which genetic changes may alter the appearance of a flu virus, saving researchers the cost of performing specialized experiments.
The group set out to discover whether predictive models that combine the genetic sequence of haemagglutinin with other experimental data could better forecast flu virus strains.
“The influenza research community has long recognized the importance of taking into account physical characteristics of the flu virus, such as how haemagglutinin changes over time, as well as genetic information,” said lead author John Huddleston, a PhD student in the Bedford Lab at Fred Hutchinson Cancer Research Center and Molecular and Cell Biology Program at the University of Washington.
“We wanted to see whether combining genetic sequence-only models of influenza evolution with other high-quality experimental measurements could improve the forecasting of the new strains of flu that will emerge one year down the line.”
Researchers looked at different components that indicate how likely the virus is to thrive and continue to evolve. These components included how similar the antigens of the virus are to previously circulating strains, how many mutations the virus has accumulated, and whether these mutations are beneficial or harmful.
Using 25 years of historical flu data, researchers made forecasts one year into the future from all available flu seasons. Each forecast predicted what the future virus population would look like using the virus’ genetic code, the experimental data, or both.
The team then compared the predicted and real future populations of flu to find out which data types were more helpful for predicting the virus’ evolution.
The results showed that forecasts that combined experimental measures of the virus’ appearance with changes in its genetic code were more accurate than forecasts that used the genetic code alone.
Models were most informative if they included experimental data on how flu antigens changed over time, the presence of likely harmful mutations, and how rapidly the flu population had grown in the last six months.
“Genetic sequence alone could not accurately predict future flu strains – and therefore should not take the place of traditional experiments that measure the virus’ appearance,” Huddleston said.
The new forecasting tool developed by researchers is open source, enabling teams from around the world to start using it to improve predictions right away.
“By releasing our framework as an open source tool based on modern data science standards like tidy data frames, we hope to encourage continued development of this tool by the influenza research community,” researchers said.
“We additionally anticipate that the ability to forecast the sequence composition of populations with earth mover’s distance will enable future forecasting research with pathogens whose genomes cannot be analyzed by traditional phylogenetic methods including recombinant viruses, bacteria, and fungi.”
With flu season looming closely on the horizon – and with the current pandemic exacerbating the potential for poor outcomes among patient populations – researchers have increasingly sought to apply data analytics tools to better predict flu trends.
In March, a team from the University of Massachusetts Amherst developed a portable tool that uses machine learning and real-time data to monitor flu-like illnesses and flu patterns.
“We are trying to bring machine learning systems to the edge,” said Forsad Al Hossain, PhD student and lead author of the study. “All of the processing happens right here. These systems are becoming cheaper and more powerful.”
The team of researchers who conducted the eLife study are confident that their findings and predictive model will help enhance flu strain forecasting for researchers around the world.
“Our results highlight the importance of experimental measurements to quantify the effects of changes to virus’ genetic code and provide a foundation for attempts to forecast evolutionary systems,” said senior author Trevor Bedford, Principal Investigator at the Vaccine and Infectious Disease Division, Fred Hutchinson Cancer Research Center.
“We hope the open-source forecasting tools we have developed can immediately provide better forecasts of flu populations, leading to improved vaccines and ultimately fewer illnesses and deaths from flu.”