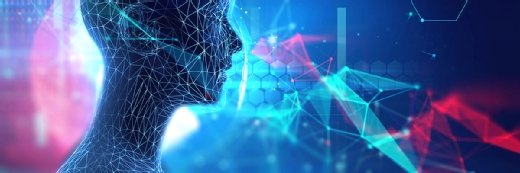
Getty Images
Deep Learning Model Could Enhance Cancer Precision Medicine
Using a deep learning model, researchers could discover the best possible drug treatments for malignant tumors and accelerate cancer precision medicine.
A deep learning algorithm was able to accurately analyze tumor data and recommend the best possible treatments for patients, potentially accelerating cancer precision medicine, according to a study published in Cancer Cell.
Just four percent of all cancer therapeutic drugs under development earn final approval from the FDA, often because developers have an incomplete understanding of the mechanisms governing drug response.
"That's because right now we can't match the right combination of drugs to the right patients in a smart way," said Trey Ideker, PhD, professor at University of California San Diego School of Medicine and Moores Cancer Center. "And especially for cancer, where we can't always predict which drugs will work best given the unique, complex inner workings of a person's tumor cells."
To overcome this issue, researchers developed a deep learning model that can match tumors to the best drug combinations. The model, called DrugCell, was specifically designed so that its recommendations can be explained to human clinicians – a necessity for healthcare AI tools.
"Most AI systems are 'black boxes' -- they can be very predictive, but we don't actually know all that much about how they work," said Ideker, who is also co-director of the Cancer Cell Map Initiative and the National Resource for Network Biology.
"We need to know why that decision is made, what pathways those recommended drugs are targeting and the reasons for a positive drug response or for its rejection.”
The team trained DrugCell on more than 1,200 tumor cell lines and their responses to nearly 700 FDA-approved and experimental therapeutic drugs, totaling more than 500,000 cell line/drug pairings. Researchers also validated some of the model’s conclusions in laboratory experiments.
Using DrugCell, researchers can input data about a tumor and the system returns the best-known drug, the biological pathways that control response to that drug, and combinations of drugs to best treat malignancy.
"We were surprised by how well DrugCell was able to translate from laboratory cell lines, which is what we trained the model on, to tumors in mice and patients, as well as clinical trial data," said Brent Kuenzi, PhD, a postdoctoral researcher in Ideker’s lab.
This study adds to UC San Diego Health’s efforts to enhance precision medicine for cancer. The organization allows patients to have a biopsy of their tumor sequenced for mutations and assessed by the Molecular Tumor Board, an interdisciplinary group of experts.
In a recent study published in Nature Communications, the precision medicine treatments recommended by the board improved outcomes for cancer patients more than standard therapies.
“One of the hurdles is that every cancer patient appears to be carrying different molecular and genomic patterns despite having the same cancer type,” said Shumei Kato, MD, associate professor of medicine at UC San Diego School of Medicine and first author of the study.
“This can be challenging since we are customizing therapy based on the unique genomic pattern patients have, and thus it is difficult to predict the response. In addition, this approach requires multidisciplinary expertise as well as access to drugs or clinical trials not always available in smaller practices.”
The researchers’ ultimate goal for the DrugCell model is to get it into clinics for the benefit of patients. However, the team noted that further research is needed before the algorithm can get to this point.
"While 1,200 cell lines is a good start, it's of course not representative of the full heterogeneity of cancer," said Jisoo Park, PhD, postdoctoral researchers in Ideker’s lab.
"Our team is now adding more single-cell data and trying different drug structures. We also hope to partner with existing clinical studies to embed DrugCell as a diagnostic tool, testing it prospectively in the real world."