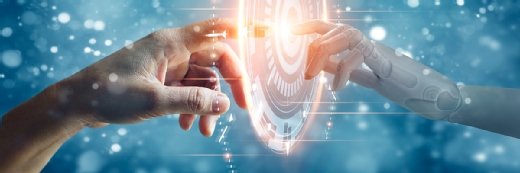
Getty Images
Machine Learning Predicts Atrial Fibrillation Hospital Readmissions
Machine learning techniques can accurately predict 90-day hospital readmissions for patients with atrial fibrillation.
A machine learning model was able to predict 90-day hospital readmissions with 85 percent accuracy, according to a study published in Health Services Research and Managerial Epidemiology.
Atrial fibrillation (AF) is a common cardiac arrythmia, causing symptoms like shortness of breath and palpitations, as well as an increase in morbidity and mortality from heart failure, stroke, and cognitive impairment.
Researchers noted that this costly condition is becoming more prevalent, with an estimated 33.5 million individuals around the world impacted by AF. In the US, the number of AF cases is expected to double by 2050, like due to the growing elderly population.
Compared to the general population, AF patients are three times more likely to undergo multiple hospitalizations, and they spend 73 percent more annually in direct medical costs. Because the number of AF cases is expected to rise in the coming years, there is an urgency around the ability to understand AF risk factors and to predict the condition.
The research team set out to use machine learning methods to predict 90-day hospital readmissions for AF patients undergoing catheter ablation. The group used data from the 2013 Nationwide Readmissions Database (NRD) on AF cases to train the machine learning algorithms, and evaluated accuracy, precision, sensitivity, specificity and area under the curve for each model.
The results show that patient’s age was the most important feature among the top 30 features selected by random forest for determination of the likelihood for being admitted.
Other top features included total number of discharges from the hospital, the number of diagnoses a patient had at discharge, the number of chronic conditions a patient had at discharge, length of initial hospital stay, and gender.
The results also showed that the machine learning algorithm achieved a predictive accuracy of approximately 85 percent, and a positive predictive value of 0.875.
The researchers stated that using machine learning methods helped identify new characteristics that may contribute to AF onset and readmissions.
“The differences in analytical methods likely attribute to the discrepancies between our research and prior research,” the team noted.
“Prior research had utilized mainly traditional statistical methods for analysis. Using machine learning to conduct analyses can lead to an improved understanding of the data and an innovative opportunity for new frontiers of discovery.”
The researchers stated that the cross-sectional nature of the NRD data was a limitation of the study, and that future research should consider collecting longitudinal data to model prediction and confirm the results.
Despite this limitation, researchers noted that the results of their study demonstrate the potential for artificial intelligence models to reduce readmissions and improve patient care.
“Such models can be valuable for both policymakers and healthcare providers. Healthcare providers might find it helpful to look closely into a patient’s record and provide patients with more personalized medical treatments to improve healthcare quality and minimize hospital readmissions,” researchers concluded.
“Applying these predictive models to assess hospital readmission risks can contribute to effective preventative treatments, lowering of medical costs, improvement in patient care, and having fewer mortalities.”
AI tools have previously shown their ability to predict hospital readmissions. A study recently published in PLOS One showed that an artificial intelligence method could reduce 30-day hospital readmissions by an estimated 12 percent, leading to lower annual healthcare costs and improved care.
The approach was also able to offer personalized treatment recommendations for patients during their hospital stay.
“The vast majority of AI in medicine develops models that learn how to predict some outcome,” said Ioannis Paschalidis, Professor of the College of Engineering (ECE, BME, SE, CDS) and Director of the Center for Information & Systems Engineering at BU.
“What is novel in this work is that we train a model that offers personalized recommendations/prescriptions aimed at improving patient outcomes. The approach has broad applicability and the potential to improve medical decision making.”