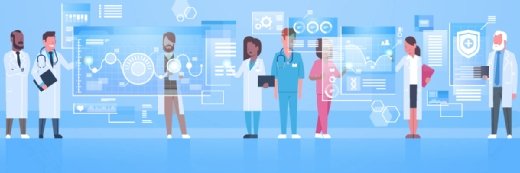
mast3r - stock.adobe.com
Predictive Analytics Model Forecasts Future Flu Outbreaks
A predictive analytics tool leverages location data to accurately forecast flu outbreaks up to 15 weeks in advance.
A predictive analytics system was able to use location data to foresee flu outbreaks, outperforming other state-of-the-art forecasting tools with an eleven percent increase in accuracy.
The tool, developed by researchers at Stevens Institute of Technology, can find patterns in the way influenza infections flow from one region to another, and use patterns identified in one region to inform predictions in other locations.
The team trained the predictive analytics algorithm using real-world state and regional data from the US and Japan. Researchers then tested the model’s forecasts against historical flu data.
While other models can use past data to forecast flu outbreaks a week or two ahead of time, incorporating location data enables much more accurate predictions over a period of several months. With the incorporation of location data, the tool can predict influenza outbreaks up to 15 weeks in advance.
The team also used a graph neural network to encode flu infections as interconnected regional clusters. This enables the algorithm to uncover patterns in infections in different places.
“Capturing the interplay of space and time lets our mechanism identify hidden patterns and predict influenza outbreaks more accurately than ever before,” said Yue Ning, an associate professor of computer science at Stevens Institute of Technology.
“By enabling better resource allocation and public health planning, this tool will have a big impact on how we cope with influenza outbreaks.”
The team also noted that the model’s predictions are explainable to humans, a critical feature for artificial intelligence in healthcare.
“Our model is also extremely transparent — where other AI forecasts use ‘black box’ algorithms, we’re able to explain why our system has made specific predictions, and how it thinks outbreaks in different locations are impacting one another,” Ning said.
Researchers noted that going forward, similar techniques could be used to predict COVID-19 surges. Although COVID-19 is a novel virus and there is no historical data to train an AI algorithm, the team pointed out that large amounts of COVID-19 location-coded data are being collected on a regular basis.
“That could allow us to train algorithms more quickly as we continue to study the COVID-19 pandemic,” Ning said.
The team is now working to incorporate additional data sources into the model to improve influenza predictions. However, accounting for current public health interventions such as vaccination education, mask-wearing, and social distancing has proven to be a challenge.
“It’s complicated, because health policies are enacted in response to outbreak severity, but also shape the course of those outbreaks,” Ning explained. “We need more research to learn about how health policies and pandemics interact.”
The team has also had to discover which data elements are truly helpful for predicting flu outbreaks and which are not.
“We’re also constrained by the information that’s publicly available,” Ning said. “Having location-coded data on vaccination rates would be very helpful, but sourcing that information isn’t easy.”
The researchers expect that the tool will be a valuable asset in real-world health planning.
“Our algorithm will keep learning and improving as we collect new data, allowing us to deliver even more accurate long-term predictions,” Ning said. “As we work to cope with future pandemics, these technologies will have a big impact.”
With the COVID-19 pandemic coinciding with flu season, researchers have sought to leverage advanced analytics tools to better predict flu trends in the US. In March 2020, a team from UMass Amherst developed a portable surveillance tool that leverages machine learning and real-time data to monitor flu-like illnesses and patterns.
The device can detect coughing sounds and crowd size in real time, helping to forecast seasonal viral outbreaks.
“Our main goal was to build predictive models at the population level, not the individual level,” said Tauhidur Rahman, assistant professor of computer and information sciences and co-author of the study.
“I thought if we could capture coughing or sneezing sounds from public spaces where a lot of people naturally congregate, we could utilize this information as a new source of data for predicting epidemiologic trends.”