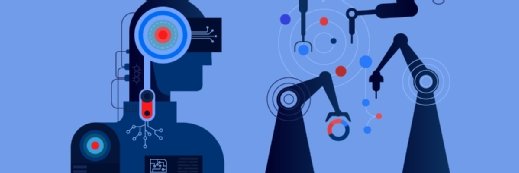
Getty Images
Predictive Analytics Model Can Improve Inpatient Sleep
A predictive analytics algorithm was able to identify which hospitalized patients do not need to be woken up overnight.
Predictive analytics could help providers determine a hospitalized patient’s overnight stability, allowing patients to rest and recover faster, according to a study published in Nature Partner Journals Digital Medicine.
Poor sleep in hospitals is a prevalent issue, with up to half of admitted patients experiencing insomnia, researchers noted. Sleep disruptions can lead to delirium, cognitive impairment, weakened immunity, hypertension, and increased mortality.
Overnight vital sign monitoring has specifically been identified as a significant cause of impaired sleep. Routine vital sign assessments occur every four to five hours for medical and surgical patients regardless of patient acuity.
Previous research has shown that targeted reductions in overnight vital sign measurements and medication administrations could result in fewer sleep interruptions and improved patient experience. However, there is no standard method of determining which patients can forgo vital sign measurements.
Researchers set out to develop a predictive analytics tool that could identify which patients don’t need to be woken up overnight. The team collected and analyzed data from multiple Northwell Health hospitals between 2012 and 2019, which consisted of 2.13 million patient visits.
They used this body of data from patient visits – including respiratory rate, heart rate, systolic blood pressure, body temperature, patient age – to develop an algorithm that predicts a hospitalized patient’s overnight stability, and whether they could be left uninterrupted overnight to sleep.
The results showed that the predictive analytics model was able to salvage approximately half of patient’s overnights in a hospital. The model’s success was achieved with relatively low-risk, researchers noted: The tool misclassified less than two out of 10,000 patient nights.
The tool also allows clinical teams to adjust the model’s predictive thresholds to implement a stricter patient assessment. To ensure proper care, researchers noted that simple visual inspection of the sleeping patients during typical nurse rounds should suffice in detecting misclassified patients, a procedure that is already part of standard nursing guidelines.
“Rest is a critical element to a patient’s care, and it has been well-documented that disrupted sleep is a common complaint that could delay discharge and recovery,” said Theodoros Zanos, PhD, assistant professor at Feinstein Institutes’ Institute of Bioelectronic Medicine.
“Our findings highlight the safety and accuracy of machine learning-based solutions to pave the way for more peaceful and safe sleep in a hospital.”
The predictive analytics model also has important implications for the nightly workload of hospital staff, as well as employee stress and burnout. Nurses spend between 20 to 35 percent of their time documenting vital signs, and approximately ten percent of their shift collecting them.
The predictive analytics tool would allow nurses to safely and confidently forgo half of the overnight vital sign measurements, and could result in up to 20 to 25 percent of workload reduction in a single overnight shift. This would enable providers to focus on more acutely ill patients.
“As healthcare systems seek to maximize efficiency and reduce waste, lean staffing models often hamper compliance with monitoring protocols as clinician capacity is exceeded, leading to delayed or incomplete care, particularly during periods of high acuity or census. Indeed, low nurse-to-patient ratios during overnight shifts have been closely linked with low protocol compliance,” researchers said.
“Our approach might also align staffing capacity and demand, positively impacting provider stress and reducing burnout, while improving operational efficiency.”
The team is currently working on implementing the predictive analytics tool in several hospitals across Northwell Health.
“Dr. Zanos and his team’s expertise in machine learning enabled them to invent an effective solution for improving sleep,” said Kevin J. Tracey, MD, president and CEO of the Feinstein Institutes. “Illness and hospitalization impair sleep cycles, and the promise for artificial intelligence in this domain holds significant promise.”