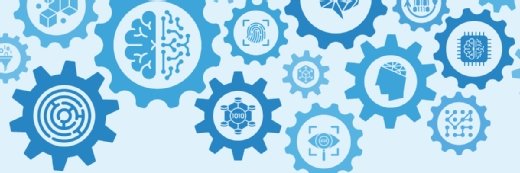
Getty Images
Machine Learning Scans Retinal Images to Predict Alzheimer’s Disease
The machine learning model analyzes retinal structure and blood vessels on images that have been correlated to Alzheimer’s disease development.
Machine learning tools can analyze certain types of retinal images to identify Alzheimer’s disease in symptomatic individuals, according to a study published in the British Journal of Ophthalmology.
Alzheimer’s disease and dementia both appear to have effects on the retina, the American Academy of Ophthalmology reports. While the most common symptoms of Alzheimer’s disease are memory loss and disorientation, symptoms can also include vision problems, issues with spatial relationships, and poor depth perception.
In the current study, researchers from Duke University developed a machine learning tool to interpret a combination of retinal images to identify patients known to have Alzheimer’s disease.
The study built on earlier work in which the team identified changes in retinal blood vessel density that correlated to changes in cognition. The group found that decreased density of the capillary network around the center of the macula in patients with Alzheimer’s disease.
With that knowledge, researchers trained a machine learning model using four types of retinal scans as inputs to teach a computer to discern relevant differences among images. The team used scans from 159 study participants to build machine learning tools. Of these participants, 123 patients were cognitively healthy, and 36 patients were known to have Alzheimer’s disease.
The results showed that combining imaging data and patient information achieved the highest level of accuracy.
“We tested several different approaches, but our best-performing model combined retinal images with clinical patient data,” said lead author C. Ellis Wisely, MD, a comprehensive ophthalmologist at Duke. “Our CNN differentiated patients with symptomatic Alzheimer’s disease from cognitively healthy participants in an independent test group.”
Researchers noted that it will be critical to enroll a diverse group of patients to build models that can predict Alzheimer’s in all racial groups as well as in those with conditions like glaucoma and diabetes, which can also alter retinal and vascular structures.
“We believe additional training using images from a larger, more diverse population with known confounders will improve the model’s performance,” said co-author Dilraj S. Grewal, MD, Duke retinal specialist.
Researchers have previously applied artificial intelligence tools to identify eye diseases in patients with chronic conditions other than Alzheimer’s. An October 2019 study showed that an AI algorithm accurately identified eye diseases in patients with diabetes 95.5 percent of the time, potentially improving care for millions of individuals who need yearly screenings for diabetic retinopathy.
“Diabetic patients already outnumber practicing ophthalmologists in the United States, and unfortunately, that imbalance is only expected to grow,” said Srinivas Sadda, MD of the Doheny Eye Institute/UCLA.
“Accurate, real-time diagnosis holds great promise for the millions of patients living with diabetes. In addition to increased accessibility, a prompt diagnosis made possible with AI means identifying those at risk of blindness and getting them in front of an ophthalmologist for treatment before it is too late.”
The findings from the current Duke-led study show that the machine learning approach could someday be used as an accessible, accurate predictive tool for Alzheimer’s disease.
“Diagnosing Alzheimer’s disease often relies on symptoms and cognitive testing,” said senior author Sharon Fekrat, MD, retina specialist at the Duke Eye Center.
“Additional tests to confirm the diagnosis are invasive, expensive, and carry some risk. Having a more accessible method to identify Alzheimer’s could help patients in many ways, including improving diagnostic precision, allowing entry into clinical trials earlier in the disease course, and planning for necessary lifestyle adjustments.”
Additional studies will determine how well the AI approach compares to current methods of diagnosing Alzheimer’s disease, which often include expensive and invasive neuroimaging and cerebral spinal fluid tests.
“Links between Alzheimer’s disease and retinal changes -- coupled with non-invasive, cost-effective, and widely available retinal imaging platforms -- position multimodal retinal image analysis combined with artificial intelligence as an attractive additional tool, or potentially even an alternative, for predicting the diagnosis of Alzheimer’s,” Fekrat said.