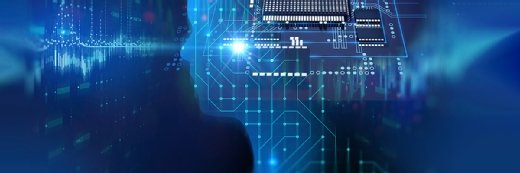
Getty Images
Deep Learning Model Speeds Analysis of Pediatric Brain Scans
The deep learning tool was able to detect enlarged cerebral ventricles on pediatric brain scans significantly faster than traditional methods.
A deep learning model can measure the volume of cerebral ventricles on pediatric brain scans, leading to improved treatment of a pathological condition called hydrocephalus, according to a study published in the Journal of Neurosurgery: Pediatrics.
Hydrocephalus is caused by an excessive amount of cerebrospinal fluid (CSF) in chambers of the brain called ventricles. The condition is the result of an imbalance between the production and absorption of CSF.
Pediatric hydrocephalus occurs in six of 10,000 live births, the researchers noted, and is known as the most common surgically correctable neurological issue in pediatric patients.
To diagnose hydrocephalus, clinicians have to identify enlarged ventricles on neuroimaging studies. Providers then insert a shunt, an internal draining system, to take excess CSF away from the brain and reduce hydrocephalus.
After this procedure, patients must be monitored periodically to make sure that the shunt is working correctly, and providers can check for changes in ventricular volume to guide decision-making. But current methods of assessing ventricular volume can be time-consuming, or require automated tools that are typically tailored to healthy adult brains and aren’t easily adapted to different patients.
The research team set out to develop a deep learning tool that could consistently assess ventricular volume over time for pediatric patients. The group also aimed to build a model that work efficiently in multiple institutions with various MRI machines from different manufacturers.
Researchers selected sets of T2-weighted MRIs from a group of 200 pediatric patients who had presented with acute obstructive hydrocephalus. For a control group, the team selected 200 sets of T2-weighted MRIs from 199 neurologically intact pediatric patients. Researchers also evaluated three-dimensional T1-weighted MRIs obtained in all control participants and a subset of patients with hydrocephalus.
The team designed the deep learning model to produce automatic ventricle segmentation and volume calculation. To evaluate the model’s effectiveness, researchers compared these two processes to the gold standard of manual segmentation and volume calculation. The team used the Dice similarity coefficient, 0 to 1, to assess segmentation accuracy.
The results showed that the deep learning model segmentation performed with an overall Dice score of 0.901 (0.946 in hydrocephalus images, 0.856 in control images). The model also generalized to external MRI images from an additional pediatric hospital with a Dice score of 0.926.
Researchers also compared the speed of the deep learning model to that of standard research software. The group found that while the standard software took 8.2 hours to 207.3 hours for ventricle segmentation and volume output, the algorithm took just 1.48 seconds per patient scan.
The results show the ability of AI tools to speed medical imaging analysis.
“By using deep learning, which does not depend on extraction of hand-crafted features, our model requires minimal preprocessing and human oversight after training is complete,” researchers stated.
“Although one prior study has demonstrated the feasibility of DL for classifying brain tissue subtypes in a small cohort of normal infants, our data set is much larger and consisted of scans from infants, children, and adolescents, some with and some without hydrocephalus.”
The team noted that the work is still preliminary, and evidence provided using the deep learning model still requires correlation with patients’ symptoms. Further study is also needed to evaluate the deep learning model when used with other types of hydrocephalus.
“Our deep learning model lays the groundwork for more widespread applications and could be used to pretrain future model iterations. Future studies could also examine model performance by using other T2 derivatives, such as single-shot spin echo or various T2-weighted imaging used for fast MRI, for even more widespread applicability,” researchers said.
The group believes that their newly developed tool can provide more accurate and timely information for improved clinical decision support.
"With near-immediate volumetric output and reliable performance across institutional scanner types, this model can be adapted to the real-time clinical evaluation of hydrocephalus and improve clinician workflow,” researchers concluded.