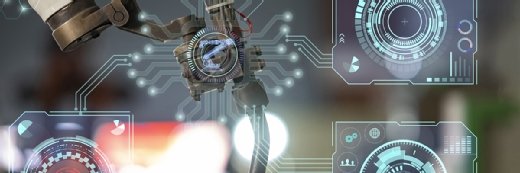
Getty Images/
Deep Learning Detects Allergic Reactions in Patient Safety Reports
Deep learning tools can identify allergic reactions from free-text descriptions in hospital patient safety reports.
A deep learning algorithm accurately identified allergic reactions in hospital patient safety reports, which could help providers avoid medical errors and improve event surveillance, according to a study published in JAMA Network Open.
Allergic reactions – to medications, foods, and healthcare products – are becoming increasingly common in the US.
Researchers noted that up to 36 percent of patients report drug allergies, and four to ten percent report food allergies. Patients in healthcare settings are at particularly high risk of developing an allergic reaction, and it’s critical that providers are able to quickly detect and monitor these events.
Clinicians can review hospital patient safety reports to get ahead of allergic reactions, but organizations often don’t have efficient ways to analyze the data in these reports.
“Manual review of keyword-filtered safety reports is time- and labor-intensive; overly sensitive parameters are associated with false-positive cases, and an overly restricted keyword repertoire is associated with missed cases,” researchers said.
The team set out to develop a deep learning tool that could identify allergic reactions in the free-text narrative of hospital patient safety reports. Researchers trained and validated the model using 101 expert-curated keywords from hospital patient safety reports at Massachusetts General Hospital (MGH).
The team then evaluated the model’s performance on three datasets: reports without keywords, reports from a different timeframe, and reports from a different hospital.
The results showed that the deep learning model achieved an area under the receiving operating curve (AUROC) of 0.979 on the dataset from MGH. The model achieved a precision of 0.930 in reports without keywords, 0.960 in reports from a different timeframe, and 0.990 in reports from a different hospital.
Researchers also found that the deep learning approach reduced the number of cases for manual review by 63.8 percent and identified 24.2 percent more cases of confirmed allergic reactions when compared with keyword-search approaches.
The findings demonstrate the potential for deep learning to identify allergic reactions and potentially improve patient safety.
“To our knowledge, this study is the first investigation that successfully used deep learning to identify allergic reactions in safety reports,” researchers stated.
“The deep learning model was able to decrease the number of cases to review during the actual case detection from a large data set and overcame the low sensitivity associated with using the keyword-search approach.”
The group made sure to develop the deep learning model so that its predictions would be interpretable to providers. When making predictions of positive cases, the model focused on words related to allergic symptoms, body locations, and common allergic reaction culprit agents. When making predictions of negative cases, the model focused on information that wasn’t relevant to allergy specifically.
The model also had a more complete list of keywords that were predictive of allergy event identification than the expert-curated list. Additionally, the model was able to enhance the expert-curated keywords by identifying misspellings, as well as offering other important keywords that were not considered by the specialists.
“These novel features enhance model transparency while augmenting the clinical knowledge base,” the team said.
Because researchers trained the model on free-text descriptions written by different providers, the algorithm could potentially extract meaningful insights from other free-text data sources like clinical notes.
“Clinical narratives in safety reports are markedly similar to clinical narratives in all free-text health care documentation. Should the model perform similarly across data types, it may be able to be used for real-time allergy detection in hospital settings,” the team said.
“After it is developed and trained, the model could detect true allergic reactions more efficiently than manual review, facilitating possible real-time applications to improve allergy documentation and clinical follow-up.”
Researchers believe that after refining their deep learning model, the tool will have critical implications for allergic reaction identification and patient safety measures.
“This study demonstrates the promise of deep learning in improving patient safety efforts with the use of automated surveillance,” researchers concluded.
“After validation on other forms of clinical data free-text description, such as clinical notes, this model could be applied to improve allergy care in healthcare settings and assessed in other patient safety domains, potentially enabling real-time event surveillance and guidance for medical errors and system improvement.”