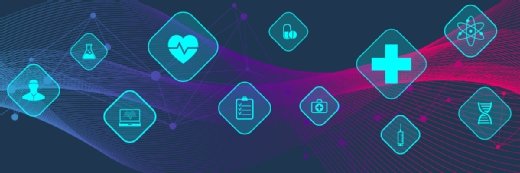
BAIVECTOR - stock.adobe.com
Machine Learning Predicts Which Breast Cancer Patients Need Surgery
The machine learning tool could help breast cancer patients avoid unnecessary treatment and reduce healthcare costs.
A machine learning algorithm can analyze medical images and predict which breast cancer patients need surgery, revealed a study published in the American Journal of Physiology – Cell Physiology.
Ductal carcinoma in situ (DCIS) of the breast – also known as stage 0 breast cancer – is a disease that only sometimes results in invasive breast cancer. Only some patients need surgery, chemotherapy, or radiotherapy, while the rest can be sent home. However, it’s difficult for clinicians to predict the outcomes with early forms of cancer.
"Typically, patients with pre-invasive cancers, such as DCIS, are treated very aggressively," said Howard Petty, PhD, a professor at the University of Michigan. "In the case of DCIS, this means partial or total mastectomies. But we know from other work that more than half of these patients will not experience invasive disease."
Researchers aimed to develop a machine learning solution that could help predict early stage cancer outcomes. The team tested the algorithm on DCIS patient samples donated to research over ten years ago, supplemented by the patients’ current medical histories.
The team stated that in DCIS cases that are going to recur as well as metastatic breast cancer, cells reorganize certain enzymes into metabolic platforms just under the outer membrane of these dangerous tumor cells.
"This allows the enzymes to operate with high efficiencies, like an assembly line at a factory," Petty said.
These efficiencies are what make those cancers dangerous. The team hypothesized that the enzyme products generated by these cellular factories promote tumor cell invasiveness and simultaneously deflect many forms of chemotherapy and radiotherapy.
To predict which DCIS cases lead to these assembly lines, researchers tagged biomarkers within patient samples and then photographed the biomarkers with a camera similar to those used in astronomy. The team then uploaded the digital images into a cloud computing platform for analysis.
“Machine learning processes images with advanced algorithms to provide information to the user; in this case, it discovered image features in addition to peripheral biomarker accumulation to classify micrographs as originating from preinvasive lesions that will lead to recurrent or nonrecurrent cancer,” researchers stated.
With this method, researchers accurately predicted cancer recurrences and non-recurrences 91 percent of the time, with just four percent false negatives.
The researchers noted that the machine learning technique will diminish the overdiagnosis of life-threatening DCIS.
“This tool would not only fit the clinical workflow but also provide rapid feedback to healthcare providers and patients. Hence, this is an attractive diagnostic tool that may be useful as a new test to identify at risk patients with DCIS,” the team said.
The group plans to further refine the tool by using larger datasets to improve its predictive power.
“In the future, it will be important to increase the number of patients studied and digital micrographs quantified to ensure that the findings can be generalized to all patients and their ducts and to improve the precision of machine learning,” the team stated.
“It will also be of interest to further explore the cell biology of recurrences, especially the identification of independent metabolic switches required for breast cancer recurrences.”
The technique may enable scientists to pharmaceutically disrupt metabolic platforms, which can block tumor invasiveness, enhance chemotherapy and radiotherapy, and stop recurrences.
"This tool may also be useful in predicting the outcomes of other pre-invasive lesions and in predicting which patients will respond to specific therapeutic interventions," Petty said.
Investigators have recently leveraged machine learning technology to enhance diagnostic capabilities and avoid unnecessary testing. A recent study conducted by researchers from Duke University showed that machine learning can analyze certain types of retinal images to identify Alzheimer’s disease in symptomatic individuals.
“Diagnosing Alzheimer’s disease often relies on symptoms and cognitive testing,” said senior author Sharon Fekrat, MD, retina specialist at the Duke Eye Center.
“Additional tests to confirm the diagnosis are invasive, expensive, and carry some risk. Having a more accessible method to identify Alzheimer’s could help patients in many ways, including improving diagnostic precision, allowing entry into clinical trials earlier in the disease course, and planning for necessary lifestyle adjustments.”