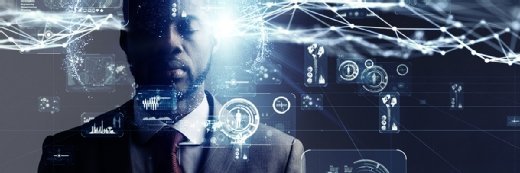
Getty Images
Machine Learning, Facebook Data Offer Insight into Schizophrenia
Using data from patients’ Facebook pages, a machine learning algorithm accurately predicted which individuals would go on to develop schizophrenia and mood disorders.
Data from sites like Facebook and Twitter can reveal a lot about someone’s behavioral health. Past studies have shown that social media activity can predict a person’s demographic characteristics, substance use, and religious and political views.
Now, researchers have applied machine learning tools to individuals’ Facebook pages in order to determine who would eventually develop schizophrenia spectrum disorders (SSD) and mood disorders – more than a year before the patient’s first hospitalization and official diagnosis.
In a new study published in Nature Partner Journals Schizophrenia, the team noted that psychiatric symptoms often emerge during adolescence or early adulthood and can interfere with the establishment of healthy social and educational foundations.
While early intervention efforts can improve outcomes for psychiatric patients, patients’ symptoms often go untreated for months or years before receiving clinical attention. Mental health professionals are looking for new ways to objectively identify early warning signs of emerging psychiatric symptoms to improve early intervention strategies.
To date, most studies focusing on the associations between social media activity and psychiatric diagnoses relied on assumptions about clinical and diagnostic status. In this study, the team set out to use real patient data with clinically confirmed and validated psychiatric diagnoses to develop machine learning algorithms – one of the first research efforts to do so.
Researchers analyzed Facebook data 18 months prior to the first psychiatric hospitalization. The group extracted 3,404,959 Facebook messages and 142,390 images across 223 consented participants, with the aim of identifying characteristics that distinguished participants with SSD and mood disorders from healthy individuals.
The results showed that people with SSD and mood disorders were more likely to use swear words than healthy participants. Individuals with SSD were also more likely to use perception words – like feel, see, and hear – than those with mood disorders and healthy people. Participants with mood disorders used more words related to blood, pain, and other biological processes.
Additionally, researchers found that the closer participants with SSD came to hospitalization, the more punctuation they used compared to healthy people, while those with mood disorders increased their use of negative emotion words.
The team also found patterns in image use among study participants: The height and width of images posted by individuals with SSD and mood disorders were smaller than those posted by healthy people, and those with mood disorders posted photos that contained more blues and less yellows.
The findings demonstrated the ability of machine learning algorithms to identify those with SSD and mood disorders using Facebook activity alone.
“There is great promise in the current research regarding the relationship between social media activity and behavioral health, and our results published with IBM Research today demonstrate that machine learning algorithms are capable of identifying signals associated with mental illness, well over a year in advance of the first psychiatric hospitalization,” said Michael Birnbaum, MD, assistant professor at Feinstein Institutes’ Institute of Behavioral Science.
“We have the potential to thoughtfully bring psychiatry into the modern, digital age by integrating these data into the field.”
Researchers pointed out that social media data, combined with AI and machine learning tools, could play a significant role in mental healthcare in the future.
“While Facebook alone is not meant to diagnose psychiatric conditions or to replace the critical role of a clinician in psychiatric assessment, our results suggest that social media data could potentially be used in conjunction with clinician information to support clinical decision-making,” researchers stated.
“Much like an X-ray or blood test is used to inform health status, Facebook data, and the insights we gather, could one day serve to provide additional collateral, clinically meaningful patient information.”
The study was limited in that some users were more active than others on Facebook, leaving researchers with varying degrees of data. The team also retrospectively collected Facebook archives to use for this analysis. In future work, researchers will need to assess how much data is needed to make a reliable clinical prediction, as well as prospectively monitor participants’ Facebook activity.
This current study builds on the research team’s previous efforts to show the potential of examining social media and online activity for psychiatry. The group recently published a paper in which they analyzed over 400,000 search queries to identify differences in timing, frequency, and content of searches among individuals with SSD, mood disorders, and healthy people.
Going forward, social media use could help mental health providers flag emerging disorders.
“Early diagnosis of serious mental illness significantly improves long term outcomes and treatment responses,” said Kevin J. Tracey, MD, president and CEO of the Feinstein Institutes. “Dr. Birnbaum is pioneering social media and digital clinical strategies to detect illness at the critical early stages when treatments are most likely to be effective.”