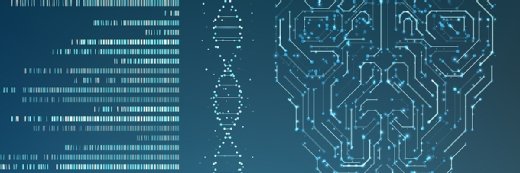
Nobi_Prizue/istock via Getty Ima
Predictive Analytics Optimizes Infectious Disease Surveillance
An early warning system leverages predictive analytics to generate more accurate near-term forecasts than alternative infectious disease surveillance methods.
A new early warning system can fill in gaps in infectious disease surveillance and forecast the spread of influenza using predictive analytics, according to a study published in Nature Communications.
Researchers noted that respiratory viruses contribute to high morbidity and mortality rates, with influenza claiming 290,000 to 650,000 lives each year alone. Surveillance and real-time forecasting systems can help prevent and control the spread of these viruses.
However, the team stated that the US Influenza Surveillance System collects data from participating outpatient healthcare clinics in all US states, Puerto Rico, the District of Columbia, and the US Virgin Islands, but not in every US county. Provider participation is voluntary, and may change over time.
Each week, approximately 3,000 providers report data to the CDC on the total number of patients seen for any reason, as well as the number of those patients with influenza-like illness.
The research team developed a forecasting model for locations without surveillance using data streams from multiple other locations collectively in a networked system informed by human movement.
The team validated the approach using historical data to demonstrate the system’s ability to forecast the spread of past outbreaks at the state and county levels. At the state level, researchers used real-world data in 35 states from the US Armed Forces Health Surveillance Branch for influenza during nine seasons (2008-2009 to 2016-2017), and human metapneumovirus and seasonal coronavirus during four seasons (2013-2014 to 2016-2017).
At the county level, the team validated their method using a model-generated outbreak since there are no historical data available in the majority of counties. The method they designed can create much more accurate near-term forecasts than alternative models tested by the researchers, including those that prioritize locations with large populations or use a random selection.
"Our goal was to design a way to provide a cost-effective early warning system so public health officials can quickly respond to outbreaks and prevent further spread," said first author Sen Pei, PhD, associate research scientist in the Department of Environmental Health Sciences at the Columbia Mailman School.
"Our method can be used to support development of a more robust surveillance system and to identify where to set up or improve surveillance."
The team noted that their approach could help forecast not just influenza, but also human metapneumovirus and seasonal coronavirus, which have similar transmission routes. Researchers could also modify the system to work with other diseases.
"Too often infectious outbreaks spread undetected due to gaps in surveillance at the community level. These gaps have contributed to tragic and unnecessary illness and loss of life, as we have seen over the past year," said senior author Jeffrey Shaman, PhD, a professor in the Department of Environmental Health Sciences at the Columbia Mailman School.
"Our method can help fill these gaps to prevent these unwanted outcomes, and provide guidance on where to invest in greater surveillance."
Researchers have previously looked to predictive analytics tools to infectious disease forecasting. In January 2019, a team from the University of Massachusetts Amherst compared the forecast accuracy of predictive analytics models to that of a historical baseline seasonal average, and found that the predictive models achieved greater accuracy than other approaches.
The team noted that their findings could further advance the field of infectious disease surveillance and forecasting.
“Our collaborative, team science approach highlights the ability of multiple research groups working together to uncover patterns and trends of model performance that are harder to observe in single-team studies. The field of infectious disease forecasting is in its infancy and we expect that innovation will spur improvements in forecasting in the coming years,” the team said.
“Public health officials are still learning how best to integrate forecasts into real-time decision making. Close collaboration between public health policy-makers and quantitative modelers is necessary to ensure that forecasts have maximum impact and are appropriately communicated to the public and the broader public health community.”