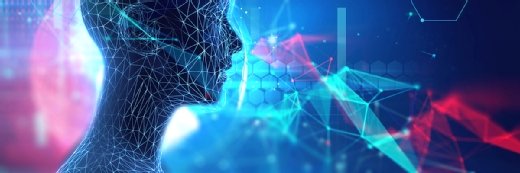
Getty Images
Deep Learning Approach Reveals Tumor Gene Expression Changes
A deep learning technique can reveal how methylation changes lead to gene expression changes in different types of tumors.
Deep learning technology can help researchers make sense of complex DNA methylation data to understand gene expression changes in tumors, study published in Genome Biology revealed.
DNA methylation data are typically used to provide biomarkers for tumor detection, subtyping, and classification, but haven’t been reliable for individual gene expression and promoter activity.
Researchers from St. Jude Children’s Hospital used data from the Pediatric Cancer Genome Project, as well as public databases such as ENCODE, to develop a deep learning framework that uses DNA methylation data to reveal promoter activity and gene expression.
The team tested the framework, called MethylationtoActivity (M2A), on a variety of adult and pediatric tumors including leukemias and solid tumors. Researchers were able to successfully decode complex DNA methylation information that reveals histone modifications, and therefore promoter and gene expression changes.
“My interest is in whether we can use deep learning to see how methylation changes are leading to promoter activity changes and gene expression changes for different types of tumors,” said corresponding author Xiang Chen, PhD, of St. Jude Computational Biology.
“What we have done with M2A is create a method for integrating DNA methylation information around promoters to make it more readily interpretable.”
The group noted that the information gathered from M2A is comparable to that obtained from ChIP-Seq, the gold standard technique for assessing promoter activity. But M2A has the added advantage of using DNA methylation data obtained from samples that aren’t candidates for ChIP-Seq because of cost or sample quality.
“It comes down to how to achieve functional interpretation of DNA methylation data, which up until now hasn’t been possible, at least to the level achieved by M2A,” said first author Justin Williams, PhD, of St. Jude Tumor Cell Biology.
“If you’ve had to choose between performing DNA methylation or doing a ChIP-Seq experiment, now you could essentially get the results of both with just DNA methylation.”
Researchers can use M2A to obtain an accurate promoter activity inference through the DNA methylome in about 15 minutes, because the M2A framework is available to scientists worldwide through St. Jude Cloud. In this platform, researchers can upload their DNA methylation data and receive a profile based on the histone modification of their choice.
Recently, deep learning tools have demonstrated their potential to improve several areas of care – most notably cancer and medical imaging.
A study published in Nature Medicine showed that a deep learning model may be able to detect breast cancer one to two years earlier than standard clinical methods. The tool also performed well when tested across populations and clinical sites not involved in training the algorithm, demonstrating promising generalizability.
“Our results point to the clinical utility of AI for mammography in facilitating earlier breast cancer detection, as well as an ability to develop AI with similar benefits for other medical imaging applications. We have developed an approach that mimics how humans often learn by progressively training the AI models on more difficult tasks,” said lead author Bill Lotter, PhD, CTO, and co-founder of DeepHealth.
“By leveraging prior information learned in each successive training stage, this strategy results in AI that detects cancer accurately while also relying less on highly-annotated data. Our approach and validation extend to 3D mammography, which is particularly important given its growing use and the significant challenges it presents for AI.”
In a separate study conducted by a team from the Center for Translational Research in Neuroimaging and Data Science (TReNDS) revealed that properly trained deep learning models could offer better insights from brain imaging data analysis than standard machine learning approaches.
“We compared these models side-by-side, observing statistical protocols so everything is apples to apples. And we show that deep learning models perform better, as expected,” said co-author Sergey Plis, director of machine learning at TReNDS and associate professor of computer science.