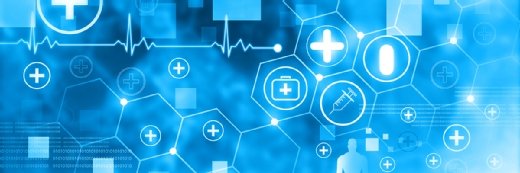
Rasi Bhadramani/istock via Getty
Data Analytics Shows Little Gain in COVID-19 Vaccine Prioritization
A data analytics model revealed that prioritizing vaccination of high-risk individuals has only a minimal impact on the number of COVID-19 deaths.
Using data analytics, a team of researchers simulated a representation of the COVID-19 pandemic in the town of New Rochelle, New York – and found that it may not be effective to prioritize high-risk individuals for vaccinations.
In a study published in Advanced Theory and Simulations, a group from the NYU Tandon School of Engineering describes the development of an open-source platform that comprises an agent-based model (ABM) for the entire town of New Rochelle.
New Rochelle was one of the first outbreaks registered in the US, the researchers noted. The size of the population in New Rochelle is also comparable to that of most American cities, making the town a crucial focal point for the study.
“We chose New Rochelle not only because of its place in the COVID timeline, but because agent-based modelling for mid-size towns is relatively unexplored despite the US being largely composed of such towns and small cities,” said Maurizio Porfiri, Institute Professor at the NYU Tandon School of Engineering.
The ABM replicates the town structure, both geographically and demographically, based on data obtained from US Census statistics. The model then superimposes a high-resolution spatial and temporal representation of the epidemic at the individual level.
The model considers physical locations as well as unique features of communities, including human behavioral trends or local mobility patterns.
To show the value of the model, researchers performed a comparative analysis of different vaccination strategies – specifically, the effect of vaccinating only high-risk groups of people versus random immunization across the entire population.
The team found that although both targeted and random approaches had some impact on COVID-19 spread, massive immunization was the only truly effective strategy. The model showed a much more significant improvement when a quarter of the town is vaccinated.
“This finding is consistent with ‘herd immunity’ predictions where effective containment of COVID‐19 can only be achieved with the large majority of the population acquiring immunity,” researchers stated.
The results also showed that even with a vaccine available, behaviors like mask-wearing, social distancing, and mobility restrictions are critical to reduce the spread of COVID-19. According to the study, the benefits of restrictive measures in place during the first wave of the pandemic greatly surpass those from any of the selective vaccination scenarios.
The team believes that this model could be invaluable for public health officials. The platform is informed by officially reported COVID-19 data, and incorporates detailed elements of pandemic spread within a statistically realistic population.
In addition to testing, treatment, and vaccination options, the model accounts for the burden of other illnesses with symptoms similar to those of COVID-19.
The model is also unique in that it can explore different testing approaches in hospitals or drive-through facilities, as well as vaccination strategies that could prioritize vulnerable groups.
“We think decision-making by public authorities could benefit from this model, not only because it is ‘open source,’ but because it offers a ‘fine-grain’ resolution at the level of the individual and a wide range of features,” said Porfiri.
The model was limited in that it did not include testing the general population, leading to possible under-detection of cases in later reopening stages. While the model allows for testing uninfected individuals with symptoms, researchers stated that massive community testing is needed to align the model’s outcomes with reality in later phases of the pandemic.
Despite its limitations, the group expects that the model could serve as an informative resource as the pandemic rages on. The World Health Organization reports that as of January 19, 2021, there are approximately 94 million cases of COVID-19 globally, and over two million deaths.
With the US in particular experiencing a massive resurgence of the virus, leaders are struggling to develop policies that will keep people safe while also preserving quality of life and local economies.
According to the researchers, models like theirs will be critical decision support tools.
“Until widespread vaccination efforts are underway, maintaining a balance between safety and normalcy during the current COVID‐19 crisis requires the use of non‐pharmaceutical prevention measures as well as efficient detection strategies,” the team stated.
“The large number of testing strategies, unknowns, and high levels of uncertainties of this epidemic calls for the principled use of predictive computational models, potentially informing policy‐making with respect to widespread vaccination efforts.”
The model may also provide leaders with valuable insights outside the pandemic.
“Beyond the timely study of vaccination strategies, our model can be adapted to explore a range of pressing problems that are ahead of us by interested users who can directly modify our open‐source platform,” researchers concluded.
“For example, the model can be swiftly adapted to describe the concurrent spread of influenza with COVID‐19, which is expected to exacerbate the impact of second and third waves. Likewise, the model can provide clear and quantitative support to the long‐debated recommendations regarding the need to avoid large gatherings and always use masks.”