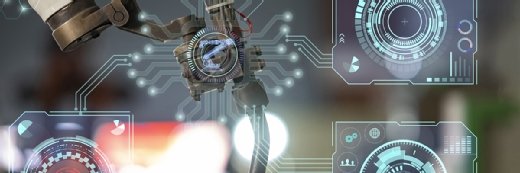
Getty Images/
Deep Learning Could Help Cancer Patients Avoid Tumor Biopsies
Using a deep learning tool, researchers were able to identify genetic mutations in tumors simply by examining 3D images of the brain.
A deep learning tool was able to identify a specific genetic mutation in a glioma tumor just by analyzing 3D brain images, with more than 97 percent accuracy.
The findings indicate that in the future, brain cancer patients may be able to avoid biopsies to help doctors determine the best treatment for their tumors. Using the deep learning model, clinicians could eliminate pretreatment surgeries in which they take glioma samples and analyze these samples to choose an appropriate therapy.
The research was conducted by a team at UT Southwestern, and presents one of the most accurate and clinically viable methods to change the paradigm of evaluating brain cancer.
"Knowing a particular mutation status in gliomas is important in determining prognosis and treatment strategies," says Joseph Maldjian, MD, chief of neuroradiology at UT Southwestern’s O’Donnell Brain Institute. “The ability to determine this status using just conventional imaging and AI is a great leap forward.”
The team used a deep learning network and standard MRI to identify the status of a gene called isocitrate dehydrogenase (IDH), which produces an enzyme that in mutated form may trigger tumor growth in the brain.
Gliomas comprise the vast majority of malignant tumors found in the brain and can often spread quickly through surrounding tissue. The five-year survival rate for high-grade gliomas is 15 percent. However, tumors with mutated IDH enzymes generally have a better prognosis.
The IDH mutation status also helps clinicians choose a combination of treatments that will be most effective for the patient, ranging from chemotherapy and radiation therapy to surgery to remove the tumor.
Doctors preparing to treat gliomas will often have patients undergo surgery to obtain tumor tissue that is then analyzed to determine the IDH mutation status. But obtaining an adequate sample can be time-consuming and risky, especially if tumors are difficult to access.
Using imaging data from a public database of more than 200 cancer patients across the US, researchers developed a deep learning algorithm that could improve the process of detecting enzyme mutations and deciding on appropriate therapies.
The model achieved a high level of accuracy after examining only a single series of MRI images, as opposed to multiple image types.
Additionally, just a single algorithm was required to assess the IDH status in the tumors. Other methods have required either hand-drawn regions of interest or additional deep learning models to identify the boundaries of the tumor and then detect potential mutations.
“The beauty of this new deep learning model is its simplicity and high degree of accuracy,” says Maldjian, adding that similar methods may be used to identify other important molecular markers for various cancers.
“We’ve removed additional pre-processing steps and created an ideal scenario for easily transitioning this into clinical care by using images that are routinely acquired.”
Researchers are planning to test and refine the deep learning model on larger datasets before incorporating the tool into clinical care. The group is also aiming to develop medications to inhibit IDH through ongoing national clinical trials. If effective, these inhibitors could combine with AI-imaging techniques to revolutionize how providers assess and treat brain tumors.
“In the big picture, we may be able to treat some gliomas without operating on a patient,” Maldjian says. “We would use AI to detect an IDH-mutated glioma, then use IDH inhibitors to slow down or reverse the tumor growth. The field of radio-genomics is exploding with possibilities.”