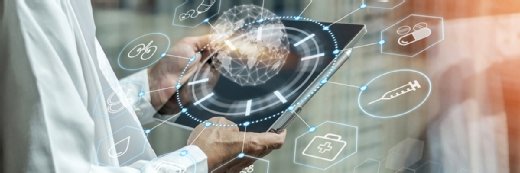
Chinnapong - stock.adobe.com
Machine Learning Identifies Existing Drugs to Treat COVID-19
A machine learning model can detect drugs already on the market that could potentially treat COVID-19.
Machine learning could help detect existing drugs that could help fight COVID-19, particularly in elderly populations, a study published in Nature Communications revealed.
One of the many major challenges of the COVID-19 pandemic has been finding effective treatments for the virus. The rapid spread of coronavirus created an immediate need for comprehensive therapies – a massive undertaking for scientists and drug developers.
"Making new drugs takes forever," said Caroline Uhler, a computational biologist in MIT's Department of Electrical Engineering and Computer Science and the Institute for Data, Systems and Society, and an associate member of the Broad Institute of MIT and Harvard. "Really, the only expedient option is to repurpose existing drugs."
Drug repurposing is an effective strategy, offering the fastest transition from research to clinical care. However, finding new uses for existing drugs requires researchers to conduct time-consuming and expensive randomized controlled trials.
Recently, investigators have explored the use of AI and machine learning to speed the drug repurposing process, with these tools accounting for hundreds or thousands of human differences within a large population that could impact how medicine works in the body.
Researchers from MIT aimed to develop a machine learning approach to identify drugs already on the market that could be potentially repurposed to fight COVID-19, particularly in the elderly.
Early on in the pandemic, it became clear that the virus caused more harm in older patients than in younger individuals on average. While it’s commonly believed an aging immune system is the reason behind this, the MIT team suggested that stiffening lung tissue could be an additional culprit.
The stiffening lung tissue shows different patterns in gene expression than in younger people, even in response to the same signal.
"Earlier work showed that if you stimulate cells on a stiffer substrate with a cytokine, similar to what the virus does, they actually turn on different genes," said Uhler. "So, that motivated this hypothesis. We need to look at aging together with SARS-CoV-2 -- what are the genes at the intersection of these two pathways?"
To identify drugs that might respond to these pathways, the researchers leveraged big data analytics technologies. Using a machine learning technique called an autoencoder, the team generated a large list of possible drugs. The group then mapped the network of genes and proteins involved in both aging and COVID-19 infection.
Researchers used statistical algorithms to understand causality in that network, allowing them to pinpoint upstream genes that caused cascading effects throughout the network. In principle, drugs targeting those upstream genes and proteins should be promising candidates for clinical trials.
The machine learning technique relied on two key datasets of gene expression patterns to generate an initial list of potential drugs. One dataset showed how expression in various cell types responded to a range of drugs already on the market, while the other showed how expression responded to infection with COVID-19.
The machine learning algorithm analyzed the datasets to highlight drugs whose impacts on gene expression appeared to combat the effects of COVID-19. To narrow the list of potential drugs, researchers focused on key genetic pathways and mapped the interactions of proteins involved in aging COVID-19 infection pathways.
The team then identified areas of overlap among the two maps, which enabled them to pinpoint the precise gene expression network that a drug would need to target to fight COVID-19 in elderly patients.
Researchers had yet to identify which genes and proteins were upstream, meaning they have cascading effects on the expression of other genes, and which were downstream, meaning their expression is altered by prior changes in the network. An ideal drug candidate would target the genes at the upstream end of the network to minimize the impacts of infection.
The team used algorithms that infer causality in interacting systems to turn their undirected network into a causal network. The final causal network identified RIPK1 as a target gene/protein for potential COVID-19 drugs, because it has numerous downstream effects. Researchers identified a list of the approved drugs that act on RIPK1 and could potentially treat COVID-19.
The team plans to share their findings with pharmaceutical companies. Before any of the drugs identified can be approved for repurpose in elderly COVID-19 patients, clinical testing is necessary to determine the efficacy of these drugs. Although this study focused specifically on COVID-19, researchers noted that their machine learning approach could be extended to other conditions as well.
"I'm really excited that this platform can be more generally applied to other infections or diseases," said Anastasiya Belyaeva, a PhD student at MIT.