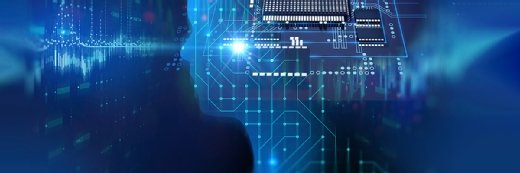
Getty Images
AI Method Facilitates Collaboration While Protecting Data Privacy
An artificial intelligence method can help researchers collaboratively train algorithms without compromising patient data privacy.
A new approach could help researchers build high-quality artificial intelligence algorithms while protecting patient data privacy, accelerating model development and innovation, according to a study published in Nature Communications.
A major challenge of developing successful AI algorithms is the availability of data and patient privacy, researchers noted. Sharing medical data, even if the information is de-identified, can pose some risk to the privacy of patients.
Recently, researchers have explored an alternative method of training AI algorithms that avoids direct data sharing. Called federated learning, the approach involves using data from a variety of institutions and distributing computational training operations across all sites.
“In federated learning, models are trained simultaneously at each site and then periodically aggregated and redistributed. This approach requires only the transfer of learned model weights between institutions, thus eliminating the requirement to directly share data,” the team stated.
Researchers from UCLA set out to demonstrate the application of federated learning at three institutions, including UCLA, the State University of New York (SUNY) Upstate Medical University, and the National Cancer Institute (NCI).
The group trained deep learning models at each participating institution using local clinical data, while they trained an additional model using federated learning across all of the institutions.
Researchers found that the federated learning approach allowed them to train AI algorithms that learned from patient data located at each of the study’s participating institutions without requiring data sharing.
The team also found that federated learning produced an AI model that worked better on data from participating institutions. Additionally, the new approach generated a model that worked better on data from different institutions than the ones that participated in the original training.
The study has significant implications for collaboration and the use of AI in healthcare.
“Because successful medical AI algorithm development requires exposure to a large quantity of data that is representative of patients across the globe, it was traditionally believed that the only way to be successful was to acquire and transfer to your local institution data originating from a wide variety of healthcare providers — a barrier that was considered insurmountable for any but the largest AI developers,” said Corey Arnold, PhD, director of the Computational Diagnostics Lab at UCLA.
“However, our findings demonstrate that instead, institutions can team up into AI federations and collaboratively develop innovative and valuable medical AI models that can perform just as well as those developed through the creation of massive, siloed datasets, with less risk to privacy. This could enable a significantly faster pace of innovation within the medical AI space, enabling life-saving innovations to be developed and used for patients faster.”
In future work, the team will aim to add an additional private fine-tuning step at each institution in order to ensure the federated learning model performs well at each institution in a large federation.
“This methodology could be applied to a wide variety of deep learning applications in medical image analysis and merits further study to enable accelerated development of deep learning models across institutions, enabling greater generalizability in clinical use,” researchers concluded.
Other organizations have leveraged federated learning to improve algorithm development and training. A study recently published in Scientific Reports showed that federated learning enables clinicians to train machine learning models while preserving patient privacy and could advance the field of brain imaging.
“The more data the computational model sees, the better it learns the problem, and the better it can address the question that it was designed to answer,” said senior author Spyridon Bakas, PhD, an instructor of Radiology and Pathology & Laboratory Medicine in the Perelman School of Medicine at the University of Pennsylvania.
“Traditionally, machine learning has used data from a single institution, and then it became apparent that those models do not perform or generalize well on data from other institutions.”