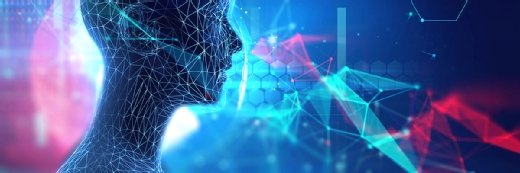
Getty Images
Machine Learning Limited When Applied to Clinical Data Registries
To fully leverage machine learning tools, the healthcare industry will need to improve the quality of clinical data registries.
When applied to a clinical data registry, machine learning algorithms showed no significant improvement in predicting adverse outcomes after an acute myocardial infarction (AMI), a study published in JAMA Cardiology.
Accurately assessing an individual’s risk of death after AMI is useful for guiding clinical decisions for patients and evaluating hospital performance. Researchers noted that existing risk prediction models developed to forecast AMI outcomes have been limited by lack of inclusion of nonlinear effects and complex interactions among variables in national samples.
“With advances in computation and analytics, however, it may be possible to create models in large and diverse patient groups, which may improve on traditional models with existing information. Specifically, the application of machine learning techniques has the potential to improve on accuracy in the prediction of in-hospital mortality after AMI,” the team stated.
Researchers used the American College of Cardiology’s (ACC) Chest Pain-MI Registry from 2011 to 2016, which includes nearly one million patients hospitalized for AMI or heart attack across more than 1,000 US hospitals.
The team applied three different machine learning models to predict death after hospitalization. The results showed that two machine learning models – gradient descent boosting and meta-classifier – only marginally improved discrimination compared with the current standard. The third algorithm, based on a neural network, showed no improvement in discriminating in-hospital mortality after AMI.
The group stated that clinical registries like the Chest Pain-MI Registry have been the mainstay for assessing patient outcomes across many hospitals through standardized data collection. While these registries can advance clinical understanding and knowledge, they are less suited for complex data collection and abstraction.
To infer additional insights, the healthcare industry may have to rethink how to aggregate novel digital data streams that are being generated at most US hospitals.
The study also highlights that while some methods are more efficient or transparent, the clinical value of machine learning will be determined by data collection and processing.
“The clinical adoption of machine learning will depend on whether it delivers better information – and that may importantly depend on the data that are used,” said Harlan Krumholz, MD, SM, director of the Center for Outcomes Research and Evaluation (CORE) at Yale and senior author of the study.
The study sheds light on the limitations of machine learning tools when applied to traditional medical databases, indicating that the way datasets are prepared may hold the key to unlocking algorithms’ value.
Machine learning techniques are well-suited for processing complex, high-dimensional data or identifying nonlinear patterns, which provide researchers and clinicians with a framework to generate new insights. Achieving the potential of AI in healthcare will require improving the data quality of EHRs.
“Our study found that advanced methods that have revolutionized predictions outside healthcare did not meaningfully improve prediction of mortality in a large national registry. These registries that rely on manually abstracted data within a restricted number of fields may, therefore, not be capturing many patient features that have implications for their outcomes,” said Rohan Khera, MD, MS, the first author of the new study.
“We believe that the next frontier for improving clinical prediction may be the application of these methods to the high-dimensional granular data collected in the EHR.”
The healthcare industry has increasingly aimed to increase and improve the use of AI tools in clinical settings. Recently, a team from UCLA leveraged a new approach to help researchers build high-quality AI algorithms while protecting data privacy, accelerating model development and innovation.
“Because successful medical AI algorithm development requires exposure to a large quantity of data that is representative of patients across the globe, it was traditionally believed that the only way to be successful was to acquire and transfer to your local institution data originating from a wide variety of healthcare providers — a barrier that was considered insurmountable for any but the largest AI developers,” said Corey Arnold, PhD, director of the Computational Diagnostics Lab at UCLA.
“However, our findings demonstrate that instead, institutions can team up into AI federations and collaboratively develop innovative and valuable medical AI models that can perform just as well as those developed through the creation of massive, siloed datasets, with less risk to privacy. This could enable a significantly faster pace of innovation within the medical AI space, enabling life-saving innovations to be developed and used for patients faster.”