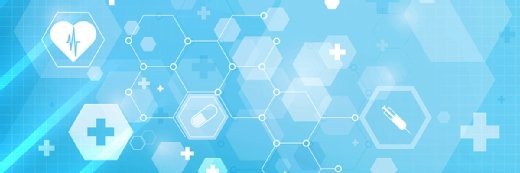
Rifqyhsn Design/istock via Getty
Machine Learning Technique Reveals Cancer Genetic Insights
Using machine learning methods, researchers discovered the underlying genetic contributors of cancer.
Machine learning approaches could help detect mutational signatures in patients with cancer, revealing the genetic effects of the underlying contributors to the disease, a study published in eLife revealed.
The new technique uses machine learning algorithms to access and analyze what are called SuperSigs, or mutational signatures that reveal the genetic effects of the underlying contributors to cancer.
“Mutational signatures are important in current cancer research as they enable you to see the signs left by underlying factors, such as aging, smoking, alcohol use, UV exposure, and BRCA inherited mutations that contribute to the development of a cancer,” said study leader Cristian Tomasetti, PhD, associate professor of oncology at the Johns Hopkins Kimmel Cancer Center.
The algorithm is classified as supervised because it is an analysis that includes known exposures during the training of the algorithm for the genetic analysis of a cancer. The most widely used mutational signatures used for assessing genomic data are classified as unsupervised because they do not take known exposures into consideration. Instead, it notes patterns and then goes back to correlate them with exposures.
The new method also allows for a mix of supervised or unsupervised approaches, controlling or blocking out the effect of known exposures to carcinogens to explore the possible effect of potential unknown factors.
Researchers found that the new supervised method outperformed the unsupervised methodology in terms of prediction accuracy. The supervised methodology had a median area under the curve (AUC) of 0.73 for aging and 0.90 for all other factors, while the unsupervised methodology had a median AUC of 0.57 for aging and 0.77 for all other factors.
“A 0.5 or below AUC means the method is not better than pure chance. The highest value you can get is 1,” said first author Bahman Afsari, PhD, an instructor at the Johns Hopkins Kimmel Cancer until a few months before publication.
The team also revealed what could be the first mutational signatures associated with cancers of obese patients, providing evidence for a mutational mechanism related to obesity and the origination of cancers.
“Obesity is arguably the most important lifestyle factor contributing to cancer, but its mechanism for causing cancer has been unknown,” said Tomasetti. “As cancers of obese patients often do not appear to have an increased number of mutations, it was thought that the mechanism through which obesity increases cancer risk was not via mutations. Our results show that it is, at least in part, mutational.”
The machine learning method also showed that an etiological, or underlying, factor does not always cause the mutational effect on all tissues, a discovery that contrasted with assumptions of the unsupervised methodology.
“Aging yields different mutational signatures in different tissues, and so do smoking and several other environmental exposures,” said co-first author Albert Kuo, Ph.D. candidate at the Johns Hopkins Bloomberg School of Public Health.
“Also, in lungs, the signature for aging and the signature for smoking are very different, but in other tissues, the signature of smoking is relatively similar to the signature for aging, suggesting inflammation as the main mechanism.”
Additionally, the research provided validation for the key role of random mutations – normal mistakes occurring within the DNA of cells during replication – in the development of a cancer.
“Every time a cell divides, it has to duplicate its DNA. As the duplication and repair machinery copies the billions of letters--the molecules that make up our DNA--mistakes are made. It is estimated that there are between three to six DNA mutations occurring every time a cell divides,” said Tomasetti.
“A major source of the mutations that cause cancer appears to be these endogenous processes that have nothing to do with genetic defective genes or harmful exposures.”
With the algorithm, the team determined that 69 percent of the mutations found in cancer patients across all tumor types can be attributed to randomly occurring mutations, indicating the need for a greater focus of effort and resources on early detection.
“If we can’t avoid cancer from occurring, then the next best thing is to find it before it is too late. If we can find a cancer at an early stage, then typically, you can save the life of the patient,” Tomasetti said.