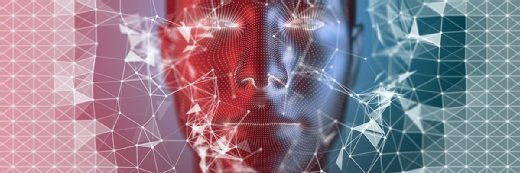
Getty Images
Artificial Intelligence Enhances Preventive Care, Telehealth
Researchers are leveraging artificial intelligence to improve preventive care measures and telehealth practices.
While preventive care and telehealth have long been critical elements of the healthcare industry, these services have become even more important in the context of the COVID-19 pandemic. Researchers are increasingly using artificial intelligence to enhance these methods of care delivery, potentially leading to improved patient outcomes.
A team from MIT has developed a deep learning algorithm that can quickly identify and screen for early-stage melanoma, a type of malignant tumor responsible for more than 70 percent of skin cancer-related deaths worldwide.
Providers have relied on visual inspection to identify suspicious pigmented lesions (SPLs), which can be an indication of skin cancer. Early-stage identification of SPLs in primary care settings can improve melanoma prognosis and significantly reduce treatment costs.
However, quickly finding and prioritizing SPLs is difficult because of the high number of pigmented lesions that providers need to evaluate for potential biopsies.
“Early detection of SPLs can save lives; however, the current capacity of medical systems to provide comprehensive skin screenings at scale are still lacking,” said Luis R. Soenksen, a postdoc and a medical device expert currently acting as MIT’s first Venture Builder in Artificial Intelligence and Healthcare.
Researchers trained an SPL analysis system using 20,388 wide-field images from 133 patients at the Hospital Gregorio Maranon in Madrid, as well as publicly available images. The images were taken with a variety of ordinary cameras that are readily available to consumers. Dermatologists working with the researchers visually classified the lesions in the images for comparison.
The team found that the system achieved more than 90.3 percent sensitivity in distinguishing SPLs from nonsuspicious lesions, skin, and complex backgrounds by avoiding the need for time-consuming individual lesion imaging.
In addition, the research presents a new method to extract intra-patient lesion saliency – the comparison of the lesions on the skin of one individual that stand out from the rest – on the basis of deep convolutional neural network (DCNN) features from detected lesions.
The findings could enable more rapid and accurate assessments of SPLs and earlier treatment of melanoma.
“Our research suggests that systems leveraging computer vision and deep neural networks, quantifying such common signs, can achieve comparable accuracy to expert dermatologists,” Soenksen said. “We hope our research revitalizes the desire to deliver more efficient dermatological screenings in primary care settings to drive adequate referrals.”
At the University of Washington, researchers are working to develop a machine learning method that uses the camera on a person’s smartphone or computer to take their pulse and respiration signal from a real-time video of their face.
“Machine learning is pretty good at classifying images. If you give it a series of photos of cats and then tell it to find cats in other images, it can do it,” said lead author Xin Liu, a UW doctoral student in the Paul G. Allen School of Computer Science & Engineering.
“But for machine learning to be helpful in remote health sensing, we need a system that can identify the region of interest in a video that holds the strongest source of physiological information — pulse, for example — and then measure that over time.”
The team first presented this innovative system at the Neural Information Processing Systems conference, and is now aiming to build a better system to measure these signals. The new system is less likely to be confused by different cameras, lighting conditions, or facial features like skin color.
“Every person is different,” Liu said. “So this system needs to be able to quickly adapt to each person’s unique physiological signature, and separate this from other variations, such as what they look like and what environment they are in.”
The system runs on the device instead of in the cloud, preserving patients’ privacy. It uses machine learning to capture subtle changes in how light reflects off of a person’s face, which correlates to changing blood flow. The system then converts these changes into both pulse and respiration rate.
Researchers trained the first version of the system with a dataset that contained videos of people’s faces and ground truth information – each person’s pulse and respiration rate measured by standard instruments in the field.
While the system worked well on some datasets, it still struggled with others that contained different people, backgrounds, and lighting. The team improved the system by having it generate a personalized machine learning model for each person. Specifically, it looks for important areas in a video frame that likely contain physiological features correlated with changing blood flow in a face under different contexts, like different skin tones, lighting, and changing environments.
Although the newer system outperformed the older one when given more challenging datasets, the team noted that the tool still needs some improvement.
“We acknowledge that there is still a trend toward inferior performance when the subject’s skin type is darker,” Liu said. “This is in part because light reflects differently off of darker skin, resulting in a weaker signal for the camera to pick up. Our team is actively developing new methods to solve this limitation.”
The team is working on a range of collaborations with providers to evaluate how this system performs in the clinic.
“Any ability to sense pulse or respiration rate remotely provides new opportunities for remote patient care and telemedicine. This could include self-care, follow-up care or triage, especially when someone doesn’t have convenient access to a clinic,” said senior author Shwetak Patel, a professor in both the Allen School and the electrical and computer engineering department.
“It’s exciting to see academic communities working on new algorithmic approaches to address this with devices that people have in their homes.”