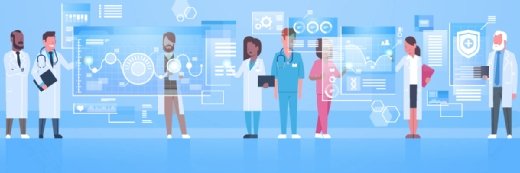
mast3r - stock.adobe.com
Big Data Framework Offers Mapping Method for Future Pandemics
A new big data framework leverages epidemiological and socioeconomic risk factors to identify populations vulnerable to COVID-19 and other infectious diseases.
A big data framework combining epidemiological and socioeconomic risk factors revealed that COVID-19 risk increased in areas with more crowding, population mobility, and morbidity, while risk decreased after the deployment of effective public health interventions.
The framework, described in a study published in the International Journal of Environmental Research and Public Health, offers a globally replicable model for mapping future pandemics.
Research conducted during and after less severe pandemics, like severe acute respiratory syndrome (SARS) and H1N1, has shown that there was a gap in how these illnesses were detected and treated among different populations. Socioeconomically disadvantaged populations were more significantly impacted by these diseases than their more affluent counterparts.
Because of these earlier studies, researchers expected that there was potential to reduce the COVID-19 outbreak by deploying targeted interventions. While more recent studies provided COVID-19 risk factors, none had identified COVID-19-vulnerable areas associated with socioeconomic status and epidemiological factors specific enough to the virus.
"The previous studies used socioeconomic status variables based on researchers' preferences, irrespective of their COVID-19 relevance. Consequently, the socioeconomic status measures across these studies were incomparable, limiting their usefulness," said Yoonjung Lee, PharmD, PhD, a pharmacy preceptor and pharmaceutical sciences researcher at the Texas Tech University Health Sciences Center (TTUHSC) Jerry H. Hodge School of Pharmacy.
For their study, the research team identified and used seven specific socioeconomic and epidemiological factors – including healthcare access, health behavior, crowding, area morbidity, education, social distancing measures, and population mobility – using South Korean incidence data.
The team filtered the study’s variables by using a conceptual mechanistic framework that describes the potential causes of disparities in the US during a pandemic. The framework attributes those disparities to varying levels of exposure to the virus, differences in susceptibility to the disease, and variations in access to healthcare.
"That directed us to causally relevant variables to COVID-19, as they determine the likelihood of being exposed to the virus agent, of contracting the disease upon exposure and of receiving timely and effective treatment after the disease has developed," Lee said.
This helped researchers design a universal definition of socioeconomic status and choose socioeconomic status indicators that were causally relevant to COVID-19 health outcomes. This methodological framework enabled researchers to identify COVID-19-vulnerable areas through their associated socioeconomic status and epidemiological elements.
"Through this approach, we could have universal socioeconomic status variables with acceptable generalizability and methodological capacity," Lee said. "Consequently, this helped make our study's regression model stronger and more accurate by using socioeconomic status variables that were relevant to COVID-19."
The findings showed that the risk of COVID-19 increased with higher area morbidity, risky health behaviors, crowding, and population mobility. Education, lower levels of social distancing, and the ability to access care also influenced risk.
Researchers also found that falling COVID-19 risks and spatial shifts over three consecutive time periods – early-phase, middle-phase, and late-phase – reflected effective public health interventions in South Korea.
"This finding can be different if other data is used, though the South Korean data, based on our knowledge, was the most detailed and publicly available data with open access, which was the reason why we used South Korean data in our study," Lee said.
"Therefore, as depicted in our study, it is crucial to identify COVID-19-vulnerable locales associated with SES and COVID-19 specific epidemiological factors and then to target prompt and effective public health interventions toward these locales for effective pandemic control."
The research is significant because it can provide leaders with a methodological framework and precision mapping method that is globally replicable for COVID-19 and future pandemics. The study provides comprehensive socioeconomic status measures that other investigators can use in future research.
"Firstly, the combined use of global and spatial statistical methods increased the accuracy as global models verified the geographical model," Lee said.
"Secondly, we collaborated in this study with researchers who developed the negative binomial extension of the geographically weighted regression. The application of this method eliminated the tool's erroneous use while also optimizing the model to study the data. Lastly, our study showed the progression of the COVID-19 epidemic over three consecutive time-periods, which was a novel approach at the time of the study."