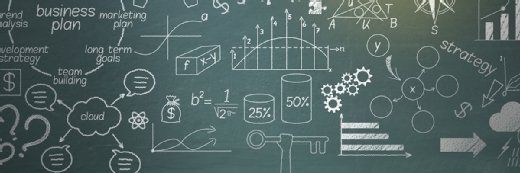
dragonstock - stock.adobe.com
FDA Evaluations of Medical AI Devices Show Limitations
Researchers reviewed approved medical AI devices and found that FDA evaluations are often retrospective and are not typically conducted in multiple clinical sites.
To ensure medical AI devices are effective, reliable, and safe, FDA evaluations should include prospective studies and assessments in multiple clinical sites, according to a review published in Nature.
With healthcare organizations increasingly looking to apply artificial intelligence algorithms to care delivery, there is a heightened need for industry-wide standards and safeguards.
“Although the academic community has started to develop reporting guidelines for AI clinical trials, there are no established best practices for evaluating commercially available algorithms to ensure their reliability and safety,” the researchers stated.
“The path to safe and robust clinical AI requires that important regulatory questions be addressed.”
The FDA has taken steps to advance its management of AI medical software. In January 2021, the agency released its Artificial Intelligence/Machine Learning (AI/ML)-Based Software as a Medical Device (SaMD) Action Plan, a response to stakeholder feedback on its 2019 regulatory framework for AI and ML-based medical products.
“This action plan outlines the FDA’s next steps towards furthering oversight for AI/ML-based SaMD,” Bakul Patel, director of the Digital Health Center of Excellence in the Center for Devices and Radiological Health (CDRH), said when the action plan was released.
“The plan outlines a holistic approach based on total product lifecycle oversight to further the enormous potential that these technologies have to improve patient care while delivering safe and effective software functionality that improves the quality of care that patients receive. To stay current and address patient safety and improve access to these promising technologies, we anticipate that this action plan will continue to evolve over time.”
Researchers on the Nature review set out to understand how the FDA is addressing issues of test-data quality, transparency, bias, and algorithm monitoring in practice. The team aggregated 130 AI devices approved by the FDA between January 2015 and December 2020.
For each algorithm, researchers assessed the number of patients enrolled in the evaluation study; the number of sites used in the evaluation; whether the test data were collected and evaluated retrospectively or prospectively; and whether stratified performance by disease subtypes or across demographic subgroups was reported.
The review showed that 126 of the 130 AI devices underwent only retrospective studies at their submission. None of the 54 high-risk devices were evaluated by prospective studies.
For most devices, researchers found that the test data for the retrospective studies were collected from clinical sites before evaluation. Additionally, the endpoints measured did not include a side-by-side comparison of clinicians’ performances with and without AI.
“More prospective studies are needed for full characterization of the impact of the AI decision tool on clinical practice, which is important, because human–computer interaction can deviate substantially from a model’s intended use. For example, most computer-aided detection diagnostic devices are intended to be decision-support tools rather than primary diagnostic tools,” researchers stated.
“A prospective randomized study may reveal that clinicians are misusing this tool for primary diagnosis and that outcomes are different from what would be expected if the tool were used for decision support.”
The review also showed that among the 130 AI devices analyzed, 93 devices did not have publicly reported multi-site assessment included as part of the evaluation study. Of the 41 devices with the number of evaluation sites reported, four devices were evaluated in only one site and eight devices were evaluated in only two sites.
“This suggests that a substantial proportion of approved devices might have been evaluated only at a small number of sites, which often tend to have limited geographic diversity,” researchers noted.
The team stated that in the past five years, the number of approvals for AI devices has increased rapidly. Over 75 percent of approvals came in the past two years and over 50 percent came in the past year. However, the proportion of approvals with multi-site evaluation and reported sample size has remained the same during that time period.
Moreover, the group pointed out that the published reports for 59 devices did not include the sample size of the studies. Of the 71 device studies that did have this information, the median evaluation sample size was 300. Just 17 device studies reported that demographic subgroup performance was considered in their evaluations.
“Although the number of sites used in a study is available to the FDA, it is also important that this information be consistently reported in the public summary document in order for clinicians, researchers, and patients to make informed judgments about the reliability of the algorithm,” researchers wrote.
“Multi-site evaluations are important for the understanding of algorithmic bias and reliability, and can help in accounting for variations in the equipment used, technician standards, image-storage formats, demographic makeup, and disease prevalence.”
In order for AI to positively impact care delivery and patient outcomes, researchers said the FDA will need to overcome these limitations in its evaluation processes.
“Evaluating the performance of AI devices in multiple clinical sites is important for ensuring that the algorithms perform well across representative populations. Encouraging prospective studies with comparison to standard of care reduces the risk of harmful overfitting and more accurately captures true clinical outcomes,” the team concluded.
“Post-market surveillance of AI devices is also needed for understanding and measurement of unintended outcomes and biases that are not detected in prospective, multi-center trials.”