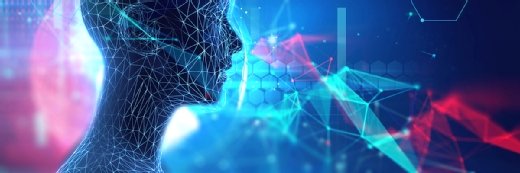
Getty Images
Predictive Analytics Tool Accurately Detects Patient Deterioration
The predictive analytics algorithm significantly outperformed existing products when identifying patients in need of life-saving interventions.
Researchers from Michigan Medicine have developed a predictive analytics model that can accurately identify patient deterioration for both general ward and COVID-19 patients.
The algorithm was more accurate than the Epic Deterioration Index (EDI), an existing tool used for patient deterioration investigation.
In a study published in JMIR: Medical Informatics, researchers describe the development and performance of the Predicting ICU Transfer and other Unforeseen Events (PICTURE) algorithm.
The team trained and validated the PICTURE model on a cohort of hospitalized COVID-19 patients using EHR data from 2014 to 2018. Researchers then applied the model to two test sets, including non-COVID-19 patients from 2019 and COVID-19-positive patients in 2020.
The predictive analytics algorithm is able to analyze a range of data, from vital signs and lab results to demographic information. Using this data, PICTURE is able to flag patients at highest risk of decline, as well as explain what risk factors influence the prediction. This can help clinicians respond faster.
“The PICTURE model is able to integrate data from the electronic health record and transform it into meaningful predictions based on the patient’s risk of experiencing an adverse outcome,” said Brandon Cummings, a data scientist at MCIRCC.
“This is especially important in the case of COVID-19 patients, who can deteriorate rapidly and unexpectedly. By predicting these events before they occur, PICTURE can give clinicians time to react and stabilize the patient before more drastic measures are required.”
Researchers found that in non-COVID-19 general ward patients, the PICTURE model achieved an area under the receiver operating characteristic curve (AUROC) of 0.819 per observation, compared to the EDI’s AUROC of 0.763.
In patients testing positive for COVID-19, PICTURE achieved an AUROC of 0.849 compared with EDI’s AUROC of 0.803.
The study was the first PubMed indexed paper to report a direct, head-to-head comparison with Epic’s EDI for COVID-19 patients. Michigan Medicine researchers are working to test PICTURE in other health systems and develop specialized versions of it for other populations, including rehabilitation and sepsis patients.
“This analysis is limited to a single academic medical center, and its generalizability to other healthcare systems will require future study,” researchers stated.
“The PICTURE model is more accurate in predicting adverse patient outcomes for both general ward patients and COVID-19 positive patients in our cohorts compared to the EDI.”
With COVID-19 cases continuing to surge and wane, researchers believe the PICTURE tool could serve as a valuable resource for ICU staff making triage decisions.
“The ability to anticipate these events will be valuable when considering potential future waves of COVID-19 infections,” says Kevin Ward, MD, executive director of MCIRCC. “However, the real value will be the continued use of PICTURE in all hospitalized patients no matter what the situation is.”
This work adds to Michigan Medicine’s efforts to leverage predictive analytics to stay ahead of poor patient outcomes. Recently, researchers from the Michigan Center for Integrative Research in Clinical Care (MCIRCC) partnered with Fifth Eye to develop the Analytic for Hemodynamic Instability (AHI), a predictive analytics tool that uses real-time streaming data to alert providers which patients are at high risk of deteriorating.
“Hemodynamic instability can happen irrespective of a patient’s condition. Whether a patient is hemorrhaging because of an injury, or a patient is septic or has some sort of infection, these kinds of things can lead to hemodynamic instability,” Ashwin Belle, PhD, former analytics architect at MCIRCC and chief analytics officer and cofounder at Fifth Eye, told HealthITAnalytics.
“Today, clinicians have very few tools in their hand to be able to see this coming. It can come on really quickly for some patients, while it takes much longer for others. To be able to see that this is happening at the onset is extremely important.”