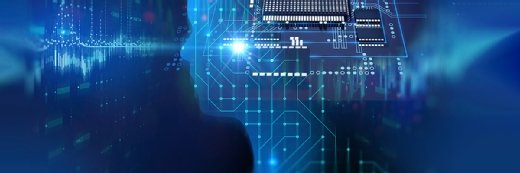
Getty Images
Predictive Analytics Model Forecasts Disease Spread, Trends
The predictive analytics model could serve as an alternative to contact tracing for anticipating disease spread.
A predictive analytics model can forecast where disease will spread from an outbreak, in what patterns, and how quickly, according to a study published in Proceedings of the National Academy of Science.
Early in the COVID-19 pandemic, public health leaders viewed contact tracing as the most effective way to anticipate the virus’s migration from the initial, densely populated hot spots to try to curb its spread. Months later, infections were nonetheless recorded in similar patterns in nearly every region of the country, both urban and rural.
A team of environmental engineers from the New Jersey Institute of Technology (NJIT) sought to find a new way to describe what was happening on the ground that doesn’t require gathering information on individuals’ movements or contacts.
Using the wealth of data published regularly by county health agencies throughout the pandemic, researchers built a predictive analytics model that could forecast and track disease spread.
“Our model should be helpful to policymakers because it predicts disease spread without getting into granular details, such as personal travel information, which can be tricky to obtain from a privacy standpoint and difficult to gather in terms of resources,” said Xiaolong Geng, a research assistant professor of environmental engineering at NJIT who built the model and is one of the paper’s authors.
The model offers an alternative way to track disease spread across the country.
“We did not think a high level of intrusion would work in the United States so we sought an alternative way to map the spread,” said Gabriel Katul, the Theodore S. Coile Distinguished Professor of Hydrology and Micrometeorology at Duke University and a co-author.
The team’s numerical scheme mapped the classic SIR epidemic model, which includes computations based on a division of the population into groups of susceptible, infectious, and recovered people. Researchers mapped this model onto the population agglomeration template. Their calculations closely approximated the multiphase COVID-19 epidemics recorded in each US state.
“Ultimately, we’d like to come up with a predictive model that would let us determine what the likely outcome would be if a state took a (particular) action,” Katul said.
By plotting on a map all of the data published weekly by county health agencies, researchers also discovered that the disease spread out across the country in similar patterns, from the largest cities to the smallest communities.
While high-infection hotspots interspersed within regions where infections remained sporadic were common early in the pandemic, the spatial signature of the infection evolved to affect most regions equally, researchers noted.
“We wondered whether the spread – in space and time – was predetermined, or if there were spatial variability related to local policy,” said Elie Bou-Zeid, a professor of Civil and Environmental Engineering at Princeton University and a co-author. “We found that the distribution tended to be proportional to the population.”
When lockdown measures began in March 2020, the virus was already out of control.
“The virus could not be isolated. While the superhighways of contagion – air flights – were curtailed, the disease spread at the local level from city to city,” said Michel Boufadel, professor of environmental engineering, director of the Center for Natural Resources at NJIT, and the corresponding author of the study.
“Using the standard precautions of masking and distancing is not enough if there are a lot of people out there. There will still be superspreader events, resulting from five to ten people gathering.”
The model allowed researchers to separately examine the two key driving mechanisms of the pandemic: Individuals taking precautions like social distancing and wearing masks, and local and state policies shutting down or reopening public spaces.
“In this case, states did not learn much from each other and that made it difficult to have a different trend,” Bou-Zeid noted.