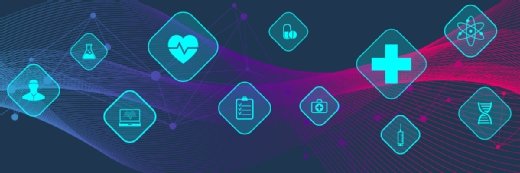
BAIVECTOR - stock.adobe.com
Deep Learning Model Predicts COVID-19 Surges 7 Days into the Future
A deep learning model uses existing big data to forecast increases in COVID-19 cases at the county level.
A deep learning tool can predict surges in COVID-19 cases for each US county with 64 percent accuracy, which is twice the accuracy of an untrained model, according to a study published in IEEE Access.
For more coronavirus updates, visit our resource page, updated twice daily by Xtelligent Healthcare Media.
In order to effectively deal with COVID-19 and future pandemics, the healthcare industry needs to design reliable intervention strategies and employ mitigation efforts. Developing these strategies will require effectively surveilling the evolution of disease over space and time, researchers noted, signaling the need for a method of forecasting the spread of a virus.
Because the spread of pandemics is influenced by a multitude of factors including mobility, population activities, and sociodemographic characteristics, typical epidemiological models that only account for a subset of relevant features are often insufficient.
Researchers from Texas A&M University set out to develop a deep learning model that can explain the complex relationship between a larger number of features to forecast COVID-19 surges in future days.
“We immediately realized the potential for employing artificial intelligence to complement the existing mathematical epidemiological models,” said Ali Mostafavi, associate professor in the Zachry Department of Civil and Environmental Engineering.
“We are living in the era of big data and leveraging these big data during crises is providing great opportunities for the development of models and data-driven tools to inform policies.”
The team trained the model using COVID-19 data from March through May 2019, focusing on four factors that influence the spread of disease both spatially and temporally: Population attributes (population density), population activities (e.g., adherence to social distancing guidelines), mobility (moving from more infected places to less infected ones), and disease spread attributes (such as reproduction number).
Using these data elements, researchers found that the tool was able to identify features to predict the trajectories of another time period: June 2019. The deep learning model was able to predict the growth of COVID-19 cases for each county with 64 percent accuracy, the team stated.
The model’s greatest accuracy was for seven days into the future, and the accuracy decreased the further it predicted into the future, which researchers said could be due to two possible reasons.
“One, the COVID-19 situation is highly dynamic and the behavior of people and the adaptive strategies they use change frequently. For example, mask use in the United States has increased over time, especially after the Centers for Disease Control and Prevention (CDC) advised it. So, if the model were trained on data before mask use became prevalent, it would learn trends that will not hold true after masks become more widespread,” the group noted.
“Two, the testing capacity of the United States continues to ramp up with time. As testing becomes more accessible, the trends a model may have learned earlier may not hold, as more people would be tested, resulting in more infected cases being found.”
Understanding which features of the model have the most significant effect on the increase of cases, public health officials could develop policies that target those factors. For example, if mobility is the most critical feature for a county, officials can establish stay-at-home policies.
The deep learning model can also offer insight into policies that may or may not be working. Through the study, researchers found that initial travel reduction orders were effective in that people from less populated counties traveled less to higher-populated cities. However, the extent of travel in densely populated counties did not change drastically.
The study demonstrated that the influence of features can change over time in one county and also vary from county to county. When the pandemic first hit, researchers saw that travel-related and mobility-related factors were important predictors of cases, but as time went on, they saw that features like social demographic characteristics were more important.
Ultimately, the progression and spread of a pandemic is highly variable, and public health policies should be developed specifically from county to county.
“One aspect of modeling that is helpful is not the accuracy, but evaluating what factors drive the outcomes,” Mostafavi said. “This model does not identify specific mitigation and response strategies, but it can help at different points in time to see which strategies could be effective based on various county-level features.”
Going forward, the research team will use new datasets to develop new types of models. The group is currently working on an AI-based model for city-scale surveillance to predict cases at the zip code level. The goal will be to predict the factors that influence each zip code so that officials can explore location-specific policies.
The results show that big data and deep learning have the potential to track and contain disease spread, as well as inform leaders about the best ways to mitigate the impacts of that spread.
“Significant opportunities exist using these big data and AI to contain the existing pandemic and also better prepare and mitigate the future pandemics,” Mostafavi said.