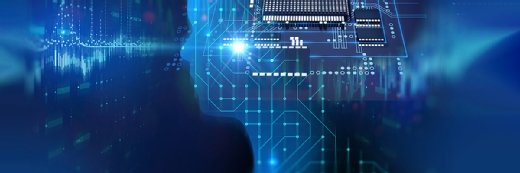
Getty Images
Machine Learning Can Streamline PTSD Diagnosis in Veterans
Machine learning could help providers diagnose PTSD more efficiently, both in veterans and the general population.
Using machine learning, researchers were able to cut six of the 20 questions used to diagnose post-traumatic stress disorder (PTSD) while still maintaining accuracy in a veteran population, according to a study published in Assessment.
PTSD impacts eight million adults in the US, the researchers stated, including hundreds of thousands of veterans of the conflicts in Iraq and Afghanistan. With the onset of the COVID-19 pandemic, PTSD symptoms are also increasing among the general population, the team noted.
However, diagnosing PTSD is time-consuming – the process typically takes 30 minutes or more, which is too long for most clinical visits.
To streamline PTSD diagnosis, researchers from the VA Boston Healthcare System and the Boston University School of Public Health (BUSPH) set out to develop a machine learning tool that would make the process more efficient.
The team used data from the Structured Clinical Interview for the Diagnostic and Statistical Manual of Mental Disorders, fifth edition (SCID-5) assessments of 1,265 veterans who served in Iraq and Afghanistan.
Researchers built a machine learning model that learned how strongly different terms in the diagnostic predicted PTSD diagnosis. This enabled the team to identify which items had weak associations and could be cut, while maintaining at least 90 percent accuracy.
For the veterans in the sample, the group found that they could cut six items: dissociative reactions, reckless or self-destructive behavior, irritable behavior and anger, hypervigilance, persistent inability to experience positive emotions, and exaggerated startle response.
"We found that several of the PTSD items could be removed because they did not make substantial contributions to accurate prediction of PTSD relative to the other PTSD items. It's likely that some of these items are removable because they are redundant with other items. Other items may be removable because they aren't specific enough to PTSD," said study lead author Tammy Jiang, a doctoral candidate in epidemiology at BUSPH.
The researchers also found that the most important item for diagnosis was detachment or estrangement from others. This was true both for the whole sample and for men and women veterans separately.
However, the results showed that different items could be cut for men and for women veterans, suggesting that providers should take different approaches when diagnosing men versus diagnosing women.
For male veterans, four items could be cut, including inability to recall important aspects of a traumatic event, dissociative reactions, reckless or self-destructive behavior, and hypervigilance.
For female veterans, items that could be cut included reckless or self-destructive behavior, dissociative reactions, persistent inability to experience positive emotions, irritable behavior and angry outbursts, exaggerated startle response, and hypervigilance.
"This study demonstrates very clearly that the most efficient manner of diagnosing PTSD may differ for men and women. This finding is especially critical in a setting like VA, which serves a small but growing number of women veterans," said study senior author Dr. Brian Marx, staff psychologist at the National Center for PTSD at the VA Boston Healthcare System and a professor of psychiatry at the BU School of Medicine.
Despite the promising results, the researchers emphasized that the machine learning algorithm won’t replace human providers, but could act as a companion tool for more efficient PTSD diagnosis.
"Our study is only a first step--but an important one, because it shows that machine learning methods can be used to help inform efforts to make care more efficient, without sacrificing or degrading the quality of care provided," said study co-author Dr. Jaimie Gradus, associate professor of epidemiology at BUSPH.
The study’s findings have significant implications for PTSD diagnosis in veterans and the general population.
"Although we already have reliable and valid screening measures for PTSD that are used in VA healthcare and other settings, they are only capable of providing provisional diagnostic determinations, which require verification using gold standard clinical diagnostic interviews," said Marx.
"Our findings potentially pave a path from which we could eventually skip right to an abbreviated--but still gold standard--diagnostic interview that would accurately identify those with PTSD and get them into treatment as soon as possible."
In the context of COVID-19, these results are even more important, researchers concluded.
"The application of machine learning methods to streamline mental health assessments may help reduce the burden, and help people receive care more efficiently," said Jiang.