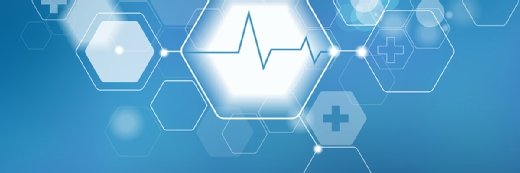
AlfaOlga/istock via Getty Images
Predictive Analytics Model Anticipates Pediatric Blood Clots
The predictive analytics model runs within the EHR to detect pediatric patients at risk of developing blood clots.
Using a predictive analytics model, providers could proactively identify pediatric patients at risk of developing blood clots or venous thromboembolisms (VTEs), according to a study published in Pediatrics.
While hospital-associated VTEs are on the rise in pediatric populations, it’s challenging for providers to identify high-risk patients. Researchers noted that case-controlled studies have resulted in general pediatric models, but few of these models have been validated.
"Hospital-associated blood clots are an increasing cause of morbidity in pediatrics," said Shannon Walker, MD, the study’s principal investigator and clinical fellow of Pediatric Hematology-Oncology at Monroe Carell Jr. Children's Hospital at Vanderbilt University.
"The reason children get blood clots is very different from adults. There was no standardized protocol for preventing clots in pediatric patients,” Walker said.
Although blood clots and VTEs are more common among adults than they are among children, the Vanderbilt team noticed that blood clot development was on the rise in pediatric populations.
“As we noticed that the rate of blood clots was going up and recognized that the adult strategy wasn't going to work for our patients, we wanted to look at each patient's individual risk factors and see how we could focus our attention on targeted blood clot prevention," Walker said.
The team built and validated a predictive analytics model that can be automated to run within the EHR of each patient admitted to the hospital.
The model includes eleven risk factors, and researchers based it on an analysis of more than 110,000 admissions to Children’s Hospital. The group then validated the tool on more than 44,000 separate admissions.
Currently, the team is studying how this model performs in conjunction with a targeted intervention in a clinical setting. The trial is called Children’s Likelihood of Thrombosis (CLOT).
For every child admitted to the hospital, the prediction model calculates a risk score. The patients are randomized, so in half of the patients elevated scores are reviewed by a hematologist. Then, each patient’s medical team and family discuss the patient’s score to determine a personalized prevention plan.
Regardless of randomization, all patients continue to receive the current standard of care.
"We are not utilizing a one-size-fits-all plan. This is an extra level of review allowing for a very personalized recommendation for each patient with an elevated score. Each day the score is updated, so as risk factors change, the scores change accordingly,” said Walker.
"We are, in real-time, assessing the use of this model as a clinical support tool. We saw a clinical opportunity of something we could improve and have moved forward with building the model -- to identify high-risk patients and are currently performing the CLOT trial, which will run through the end of the year."
The study was made possible with the help of the Advanced Vanderbilt Artificial Intelligence Laboratory (AVAIL).
"AVAIL served as a catalyst, in this instance by bringing experts in a complex trial development into proximity so that a great synthesis could happen," said Warren Sandberg, MD, PhD, executive sponsor of AVAIL.
The AVAIL program, currently in its second year, aims to support AI tools at VUMC through project incubation and curation, including facilitating clinical trials to evaluate their effectiveness.
"What is unique about this particular project is that we were not only able to predict complications but also able to test the model in a rigorous, pragmatic, randomized, controlled trial to see if it benefits patients," said Dan Byrne, senior biostatistician for the project and director of artificial intelligence research for AVAIL.
"The future of this kind of work is unlimited. We can hopefully use this approach to predict and prevent pressure injuries, sepsis, falls, readmissions or most any complication before they happen. At Vanderbilt, we are raising the bar when it comes to the science of personalized medicine and application of artificial intelligence in medicine in a way that is both ethical and safe."