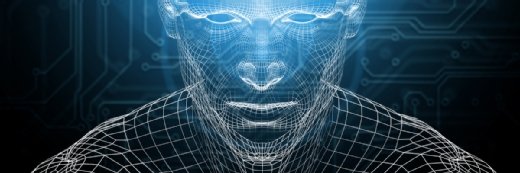
Getty Images
Deep Learning Network Predicts Adverse Cardiac Events
Researchers developed a deep learning network that can predict a patient’s risk of adverse cardiac events, including heart attacks and death, a new study reveals.
A deep learning network can predict adverse cardiac events including heart attacks and death, according to recent research presented at the Society of Nuclear Medicine and Molecular Imaging 2021 Annual Meeting and a corresponding study published in the Journal of Nuclear Medicine.
Over 20,000 patients were included in the study, split into training, validation, and testing cohorts. The deep learning network was able to predict major adverse cardiac events (MACE) using single photon emission tomography (SPECT) myocardial perfusion imaging (MPI).
Specifically, researchers used data from the largest SPECT dataset called the REgistry of Fast myocardial perfusion Imaging with NExt generation SPECT (REFINE SPECT).
Researchers also developed a mechanism that identifies polar map image regions so that physicians can easily visually interpret a patient’s risk level. The deep learning network gave each patient a score that quantified how likely they were to have a major adverse cardiac event. Patients were tracked for a follow-up period of 4.7 years on average.
"These findings show that artificial intelligence could be incorporated in standard clinical workstations to assist physicians in accurate and fast risk assessment of patients undergoing SPECT MPI scans," explained Ananya Singh, MS, a research software engineer in the Slomka Lab at Cedars-Sinai Medical Center in Los Angeles, CA, in a press release.
"This work signifies the potential advantage of incorporating artificial intelligence techniques in standard imaging protocols to assist readers with risk stratification."
The deep learning network “was developed with polar map image inputs of raw perfusion and gated derived maps of motion, thickening, phase angle and amplitude combined with age, sex, end-systolic, and end-diastolic volumes,” the study stated.
The polar map imaging enabled the network to highlight specific regions of the heart associated with MACE risk and could provide a score in less than one second. Patients in the highest quartile of scores experienced a 9.7 percent annual MACE rate, a 10.2-fold increase relative to patients with the lowest scores.
“Use of a deep learning network allows for prediction of MACE directly from polar map images with improved accuracy compared to automatic quantitation of perfusion,” the study stated, suggesting that the deep learning network was able to produce better results than current methods.
A recent study revealed that using deep learning, radiologists could assess and determine types of lung cancer on CT scans, and in turn develop precision medicine treatments. Also using deep learning along with genomic data, researchers found that a blood test may help predict a patient’s risk of Alzheimer’s disease. The deep learning algorithm revealed 152 genetic variations in those with Alzheimer’s compared to healthy patients.
Deep learning, a form of artificial intelligence, has shown promise in healthcare in recent years. From predicting to diagnosing and preventing, the speed and accuracy of deep learning networks have a multitude of clinical applications.