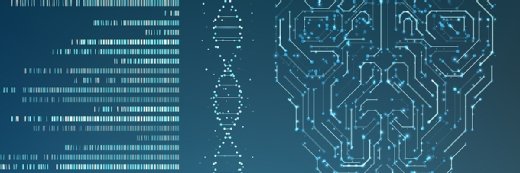
Nobi_Prizue/istock via Getty Ima
Deep Learning Aids Prediction of Lung Cancer Immunotherapy Response
Researchers developed a non-invasive method of predicting lung cancer immunotherapy response using deep learning and PET/CT images to measure PD-L1 biomarker levels.
H. Lee Moffitt Cancer Center & Research Institute researchers developed a deep learning model that uses PET/CT images to measure levels of the Programmed death-ligand 1 (PD-L1) biomarker and predict a lung cancer patient’s response to immunotherapies, according to a study published in the Journal for ImmunoTherapy of Cancer.
Researchers set out to determine whether they could predict the need for immune checkpoint inhibitors (ICIs), a drug therapy used to treat non-small cell lung cancer that only a about half of patients respond effectively to.
Currently, the clinically approved way to determine if immune checkpoint inhibitors are going to be effective is by conducting an invasive biopsy to determine PD-L1 expression status. Researchers hypothesized that using a combination of PET/CT images and deep learning algorithms could provide a non-invasive alternative for determining ICI treatment eligibility.
Moffitt researchers developed a deeply learned score (DLS) using data from 697 patients with non-small cell lung cancer to predict the PD-L1 expression status. The model also predicted the durable clinical benefit, progression-free survival, and overall survival in three cohorts of late-stage non-small cell lung cancer patients who received ICI treatments.
"These data demonstrate the feasibility of using an alternative non-invasive approach to predict expression of PD-L1," Matthew Schabath, PhD, an associate member of the Cancer Epidemiology Department at Moffitt Cancer Center, said in a press release.
"This approach could help physicians determine optimum treatment strategies for their patients, especially when tissue samples are not available or when common testing approaches for PD-L1 fail."
The deep learning method was trained using features of PET/CT scan images, including size, shape, textures, and pixel intensity. In this analysis, the computers learned how to accurately predict PD-L1 expression and later predict immune checkpoint inhibitor outcomes.
"Because images are routinely obtained and are not subject to sampling bias per se, we propose that the individualized risk assessment information provided by these analyses may be useful as a future clinical decision support tool pending larger prospective trials,” the study concluded.
Current clinical decision support methods for this particular cancer are limited to invasive, time-consuming, and potentially dangerous biopsies that are used just to determine if a patient might respond to ICIs. Deep learning enables clinicians to safely assess PD-L1 biomarker levels and determine the appropriate course of treatment.
Other advances in deep learning have shown promise for risk prediction, allowing clinicians and their patients to get ahead of potential negative health outcomes. Deep learning has the potential to mitigate risk and provide clinicians with useful clinical decision support tools that can aid treatment.
Researchers recently developed a deep learning network that can predict major adverse cardiac events and death. The deep learning network gave each patient a score that estimated how likely they were to have a major adverse cardiac event, and patients were tracked for an average of 4.7 subsequent years to determine the estimate’s validity.