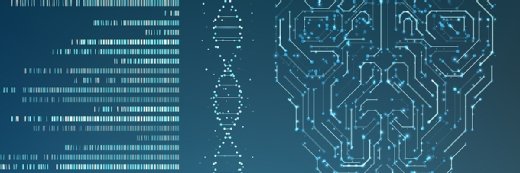
Nobi_Prizue/istock via Getty Ima
Machine Learning Algorithm Brings Predictive Analytics to Cell Study
A new machine learning algorithm system uses predictive analytics to determine which transcription factors are active in individual cells.
Scientists at the University of Illinois Chicago have introduced a new system that uses a machine learning algorithm and predictive analytics to find what transcription factors are most likely to be active in individual cells. The system was created to provide researchers with a more efficient method of identifying the regulators of genes.
Transcription factors are proteins that bind to DNA and have control around what genes are active inside a cell. Understanding and manipulating these signals in a cell is crucial to the biomedical field. Additionally, using this method of manipulating signals within a cell has proven to be an effective way to discover new treatments and illnesses.
However, there are hundreds of transcription factors inside a human cell. It could take years of research, and lots of trial and error, to determine the most active factor.
"One of the challenges in the field is that the same genes may be turned ‘on’ in one group of cells but turned ‘off’ in a different group of cells within the same organ," Jalees Rehman, UIC professor in the department of medicine and the department of pharmacology and regenerative medicine at the College of Medicine, said in a press release.
"Being able to understand the activity of transcription factors in individual cells would allow researchers to study activity profiles in all the major cell types of major organs such as the heart, brain or lungs," Rehman continued.
The system developed by the University of Illinois Chicago is named BITFAM, standing for Bayesian Inference Transcription Factor Activity Model. The machine learning algorithm system operates by “combining new gene expression profile data gathered from single cell RNA sequencing with existing biological data on transcription factor target genes,” UIC stated in a press release.
With all the information, the system will run multiple computer-based simulations to find the best fit and predict the activity for every transcription factor in the cell.
The system was tested on cells from tissue in the lung, heart, and brain by Rehman and fellow UIC researcher Yang Dai, UIC associate professor in the department of bioengineering at the College of Medicine and the College of Engineering.
"Our approach not only identifies meaningful transcription factor activities but also provides valuable insights into underlying transcription factor regulatory mechanisms," Shang Gao, first author of the study and a doctoral student in the department of bioengineering said in a press release.
"For example, if 80% of a specific transcription factor's targets are turned on inside the cell, that tells us that its activity is high. By providing data like this for every transcription factor in the cell, the model can give researchers a good idea of which ones to look at first when exploring new drug targets to work on that type of cell," Gao continued.
According to the researchers, the machine learning algorithm system is available to the public and could be applied widely. Users can combine the system with additional analysis methods that may be better suited for their own studies. This could include finding new drug targets.
"This new approach could be used to develop key biological hypotheses regarding the regulatory transcription factors in cells related to a broad range of scientific hypotheses and topics. It will allow us to derive insights into the biological functions of cells from many tissues," Dai said.
Rehman explained the application relevant to his lab is to use the new machine learning algorithm system to focus on factors that increase disease in certain cells.
“For example, we would like to understand if there is transcription factor activity that distinguished a healthy immune cell response from an unhealthy one, as in the case of conditions such as COVID-19, heart disease or Alzheimer's disease where there is often an imbalance between healthy and unhealthy immune responses," Rehman said.