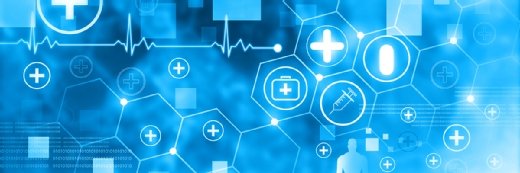
Rasi Bhadramani/istock via Getty
How Broad Data Collection Can Eliminate Health Disparities
Broad data collection can improve precision medicine, artificial intelligence, and predictive analytics to eliminate health disparities.
With the use of broad data collection, medical professionals can provide better care for their patients and eliminate health disparities. However, as health analytics advances to improve outcomes for patients, there are also opportunities for potential health inequities.
To ensure all patients are receiving equal and quality care, analytic health technology should engage in broad data collection practices. By focusing efforts on diverse data collection, these tools can improve precision medicine, artificial intelligence, and predictive analytics to eliminate health disparities.
Data Collection for Precision Medicine
The success of precision medicine relies on large databases containing information regarding genetics, environmental factors, and lifestyle choices. Clinicians can use precision medicine to determine treatment options that work best for a patient depending on that patient’s unique personal characteristics.
Not only does precision medicine require large databases, but the databases should also contain data from diverse populations to eliminate health disparities. Racial disparities in chronic diseases are well documented in the medical field.
According to an article from Nature, “Black people in the United States are around twice as likely to die of prostate or stomach cancer as their white counterparts. Black and Hispanic people are diagnosed younger and with more aggressive types of breast cancer than white people. Across most cancer types, death rates are higher for Black people than they are for other groups.”
To meet the needs of diverse populations and engage in precision medicine practices, databases should contain data that is representative of various groups of individuals, rather than predominantly one or two. One way to diversify data is to take part in data-sharing efforts.
By making more data available to multiple organizations, researchers can improve precision medicine practices and provide better care for all.
Improved Use of Artificial Intelligence
Artificial intelligence capabilities have become increasingly common in the healthcare field. AI can lighten the load of physicians and provide better care for patients. To ensure the best quality care for individuals, researchers need to gather large quantities of data that equally represents diverse populations.
While collecting sizable amounts of data will better serve diverse populations, using substantial data quantities creates more work for physicians. However, AI techniques such as deep learning allow for artificial intelligence to comb through large amounts of data and analyze the information. Physicians can then use that information to evaluate conditions and guide treatment options.
Additionally, it’s important for researchers to correctly train AI algorithms to ensure the data collection process is addressing health disparities rather than creating more. To eliminate potential algorithm bias, the Center of Applied AI at Chicago Booth’s playbook outlines four steps providers should pursue.
Developers should assess the algorithm, understand the target, update or eliminate the algorithm if it is not efficient anymore, and continue to monitor and conduct audits on the algorithm.
By collecting broad data for artificial intelligence technology, providers can use techniques to help lessen health disparities.
Big Data Enhances Predictive Analytics
Predictive analytics has proven to be a useful tool among researchers and healthcare providers. By analyzing historic data and trends, medical professions can better examine the patient’s condition and determine treatment options.
Additionally, predictive analytics can play an important role in the medical decision-making process. University of Michigan Rogel Cancer Center researchers are creating a blood test that can predict whether certain treatment methods for HPV-positive throat cancer are working months before standard imaging scans.
"Currently, the only way doctors know if a treatment is working is for the patient to get an imaging scan every few months to see whether their tumors are shrinking," oncologist Paul Swiecicki, MD said in a press release.
"And this isn't fully accurate since some cancers show what we call pseudoprogression, where a successful treatment makes the tumors bigger before it shrinks them. Our goal was to develop a test that could tell us whether a treatment is likely to work after a single cycle," Swiecicki said.
Using predictive analytics allows for providers to assess whether a treatment option will work by examining historic data or determine if a certain treatment method is working based on early signs of indication.
For predictive analytics to work effectively, researchers need to collect broad data from diverse sources. If a study collects data from populations that are proximately White, that data isn’t going to reflect the unique challenges that people of color face in the healthcare community.
Additionally, data collection should not only consist of demographic data but also socioeconomic and environmental factors and social determinants of health to eliminate health disparities.
In a study using machine learning, researchers used predictive analytics to determine the likelihood of cardiovascular disease and make treatment decisions. To get a complete understanding of someone's risk factors for chronic disease, the researchers argued that providers should be looking at patients’ full health profiles, including socioeconomic and environmental factors.
By incorporating social determinants of health into predictive analytic machine learning models, providers can develop practices to better manage chronic conditions and recommend treatment options that consider a patient’s socioeconomic and environmental factors.
Through broad data collection, researchers can develop and enhance precision medicine, artificial intelligence, and predictive analytics to provide a better quality to patient care and eliminate health disparities.