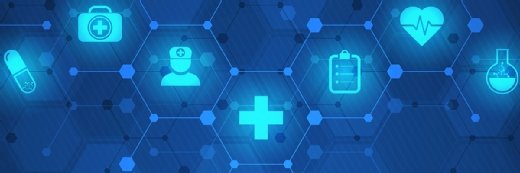
bestbrk/istock via Getty Images
Machine Learning Triage Tool Better Predicts Mortality in ED
A recent study shows how an interpretable machine learning tool can predict the mortality probability of emergency department patients.
In a cohort study, researchers compared how an interpretable machine learning triage tool for predicting mortality operates in a cohort of patients admitted to the hospital from the emergency department (ED) versus standard triage scores.
ED triage is a complex clinical judgment-based process to understand a patient’s probability of survival and availability of medical resources. While scoring tools are critical in risk stratification, current score systems have shown limitations.
“To address the need for a risk tool appropriate to the ED workflow, we developed the Score for Emergency Risk Prediction (SERP) using a general-purpose machine learning-based scoring framework named AutoScore,” research authors wrote in the study.
By using the machine learning tool, researchers could provide an accurate estimate of a patient’s risk of death. The study assessed all ED patients between January 1, 2009, and December 31, 2016.
To determine mortality outcomes after emergency admissions, SERP was compared to several other triage systems including Patient Acuity Category Scale, Modified Early Warning Score, National Early Warning Score, Cardiac Arrest Risk Triage, Rapid Acute Physiology Score, and Rapid Emergency Medicine Score.
Through the analysis, three SERP scores, SERP-2d, SERP-7d, and SERP-30d, were developed to predict mortality after 2, 7, and 30 days. Researchers then compared SERP outcomes to already existing clinical scores.
“We also tested the performance of those clinical scores on the secondary outcomes, including inpatient mortality, defined as deaths in the hospital, and 3-day mortality, defined as deaths within 72 hours after the time of admission. Death records were obtained from the national death registry and were matched to specific patients in the EHR,” the researchers wrote.
Electronic health record (EHR) data were extracted from the SingHealth Electronic Health Intelligence System. The patient data was de-identified, and comorbidities were obtained from hospital diagnosis and discharge records.
Using statistical analysis, the study indicated that SERP had a better prediction rate than existing triage scores and maintained easy implementation and ease of ascertainment in the ED. According to the researchers, this method of mortality prediction could become broadly accepted in the healthcare community.
“SERP is a parsimonious and point-based scoring tool for triaging patients in the ED. In this cohort study, SERP performed better in comparison with existing triage scores and has the advantage of easy implementation and ease of ascertainment at ED presentation.”
“SERP scores have the potential to be widely used and validated in different circumstances and health care settings. Following the clinical application of SERP in ED triage, more tailored scores can be derived in various clinical areas through the machine learning-based AutoScore framework in the future,” the authors concluded.