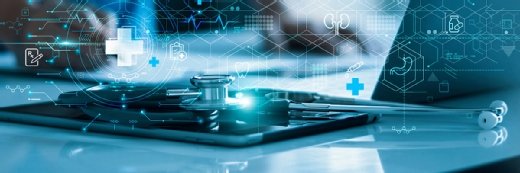
ipopba - stock.adobe.com
Predictive Model Illustrates Transmissibility of COVID-19
By analyzing similarities between the flu and COVID-19, researchers developed a predictive model to determine how COVID-19 spreads.
University of Chicago researchers used flu data and similarities to create a predictive model showing how COVID-19 spreads.
While COVID-19 is more transmissible and deadlier than most influenzas epidemics encountered in our lifetimes, COVID-19 and the flu do share some characteristics. Both illnesses primarily infect the upper respiratory system and are spread by droplets, fomites, and contact.
Professor of medicine at the University of Chicago Ishanu Chattopadhyay, PhD, and his postdoctoral scholar Yi Huang, PhD, used their previous experience modeling epidemics and expertise in machine learning to analyze years of past influenza epidemics.
The new risk measurement the team developed, the Universal Influenza-like Transmission (UnIT) score, has proven to be more accurate at predicting weekly case counts compared to current models.
“Even before COVID-19, we were working on modeling pandemics in general,” Chattopadhyay said in a press release.
“There are multiple challenges presented by a pandemic. One is thinking about how a new strain emerges, and how the virus came to be — and the other is predicting the case counts. It’s very important to understand where the disease came from, but once it’s a pandemic, it’s important to be able to predict how it’s going to move through the population and through cities in order to develop public health policies.”
Chattopadhyay, the paper’s senior author, and Huang jumped at the opportunity to use their combined skills to assist in modeling the pandemic nationwide. However, when they looked at the existing models, they noticed that the data did not investigate the similarity between COVID-19 and seasonal influenza trends.
“These are different diseases, but there are similarities in how they are transmitted. One indication of this is how the measures we took to curb the spread of COVID-19 also curbed the spread of the flu. So, the question is, can we actually use patterns of how flu spreads in the U.S. to inform this modeling of how COVID-19 spreads?” Chattopadhyay said.
The researchers used ten years of data on influenza hospitalizations nationwide to study week-to-week trends in patients with the flu, allowing them to find where infection clusters began and how they spread across the country each year. With this data, the team produced the UnIT score.
By combining other variables that impact COVID-19 spread, such as social determinants of health, the model created forecasting results that were more accurate on average than any other models listed on the CDC modeling hub.
“Our model is relatively simple, with far fewer variables than many of the other models being used to predict case counts and deaths. And yet we beat out those other, more complicated models on average over the entire pandemic timeline,” said first author Huang, now an associate research scientist at Brookhaven National Laboratory.
“This shows us that we can learn something valuable from things we already know, like influenza epidemics, and can combine that knowledge of history with principles in statistics to come up with a new and meaningful way to predict something truly unknown.”
According to researchers, these results are important in not only understanding the spread of COVID-19 but also extending to help predict future pandemic outbreaks. The algorithm developed by the researchers has been shared on the CDC COVID-19 Forecast Hub, where it can be accessed by others and is used as part of the CDC’s prediction modeling for COVID-19.