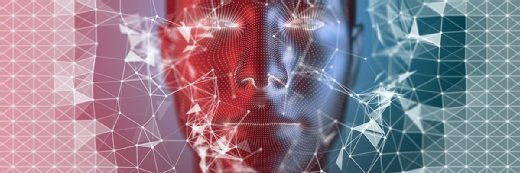
Getty Images
Artificial Intelligence Capable of Predicting COVID-19 Symptoms
Based on comorbidities, researchers can use artificial intelligence to predict which COVID-19 symptoms a patient will develop.
Georgia Institute of Technology and Emory University researchers created a new artificial intelligence-based tool to assist clinicians in predicting which symptoms COVID-19 patients will experience based on comorbidities. Additionally, the AI tool can suggest specific FDA-approved drugs for treatment.
The new tool is called MOATAI-VIR (Mode Of Action proteins & Targeted therapeutic discovery driven by Artificial Intelligence for VIRuses.) According to researchers, the artificial intelligence tool predicts 24 out of 26 major clinical manifestations of COVID-19 and the underlying disease-protein-pathway relationships.
The clinical manifestations include a range of side effects such as acute respiratory distress, blood clotting issues, cytokine storms, low blood oxygen levels, low white blood cell counts, and marrow failure. Other more common symptoms, including loss of smell or taste and “brain fog,” were also examined.
“It’s still the question of, what’s causing the side effects?” the corresponding author for the study Jeffrey Skolnick said in a press release.
“So, you lost sense of smell and got brain fog — and another (patient) had respiratory distress, and another can’t remember the day of the week. What we’ve identified are the possible mode of action drivers for these various conditions, which is now setting the stage for who’s getting what side effects.”
Skolnick also noted that using predictive analytics and artificial intelligence to determine COVID-19 side effects based on protein interactions makes sense.
“Humans are molecular machines, and presumably there are biological and physical rules to dictate our responses. We basically built an AI-based approach which was designed given the interactive set of proteins in humans which interact with the [novel] coronavirus,” Skolnick continued.
“We then asked ourselves, ‘Could we predict, based on biochemical pathways, which interactive proteins are associated with side effects?’”
According to Skolnick, most known diseases are due to the malfunction and interaction of different proteins. By identifying the protein targets of comorbidities, researchers can use predictive analytics to determine the severity and side effects of COVID-19.
The MOATAI-VIR methodology can then assist clinicians in precision medicine efforts to target the impacted protein and treat the disease. The researchers reported that the methodology had 72 percent success in 123,146 drug-indication pair predictions.
“For a given disease, we prioritize them by the proteins that are most in common with the comorbid diseases to the given disease, giving rise to the particular complication, such as respiratory failure. This identifies the putative (assumed) driver proteins for the given complication,” Skolnick said.
“Then we select repurposed drugs in two ways — we screen the most common comorbid proteins for their most frequent binding to repurposed drugs. For the set of comorbid diseases to a given complication, choose the drugs that treat the most complications.”
While using these decision tools can improve patient outcomes, Skolnick cautions that algorithms must be properly trained to achieve the desired results.