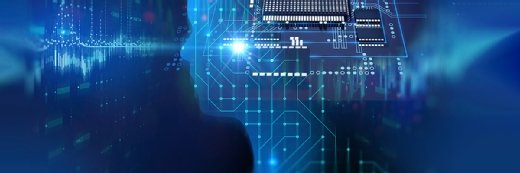
Getty Images
Machine Learning Predicts Cancer Treatment Response
Researchers are using a multi-algorithm machine learning approach to determine how cancer patients will respond to drug treatment.
The Georgia Institute of Technology and Ovarian Cancer Institute researchers are using machine learning algorithms to predict how patients will respond to cancer-fighting drugs.
Advances in machine learning and artificial intelligence are allowing researchers to create more targeted precision medicine-based treatment using predictive analytics. By analyzing large amounts of complex data, clinicians can provide individualized treatments, improving patient outcomes.
“In medicine, we need to be able to make predictions,” professor at the School of Biological Sciences and director of the Integrated Cancer Research Center in the Petit Institute for Bioengineering and Bioscience at the Georgia Institute of Technology, John F. McDonald, said in a press release.
To make medical predictions, researchers can develop their understanding of cause and reflect relationships such as how a cancer patient responds to drug treatments. However, another option is through analyzing correlation.
“In analyzing complex datasets in cancer biology, we can use machine learning, which is simply a sophisticated way to look for correlations. The advantage is that computers can look for these correlations in extremely large and complex data sets.”
For the study, McDonald and his team created predictive machine learning-based models for 15 distinct cancer types, using data from 499 independent cell lines from the National Cancer Institute.
The models were then validated with a clinical dataset containing seven chemotherapeutic drugs, administered either singularly or in combination to 23 ovarian cancer patients. The model indicated an overall predictive accuracy of 91 percent.
“While additional validation will need to be carried out using larger numbers of patients with multiple types of cancer,” McDonald noted, “our preliminary finding of 90% accuracy in the prediction of drug responses in ovarian cancer patients is extremely promising and gives me hope that the days of being able to accurately predict optimal cancer drug therapies for individual patients is in sight.”
The complexities of cancer make it difficult to predict drug responses. According to McDonald, even patients with the same types of cancer can respond differently to the same treatment.
“Part of the problem is that the cancer cell is a highly integrated network of pathways and patient tumors that display the same characteristics clinically may be quite different on the molecular level,” McDonald explained.
The goal of personalized cancer medicine is to accurately predict responses based upon genomic profiles of individual patient tumors.
“In our approach, we utilize an ensemble of machine learning methods to build predictive algorithms — based on correlations between gene expression profiles of cancer cell lines or patient tumors with previously observed responses — to a variety of cancer drugs. The future goal is that gene expression profiles of tumor biopsies can be fed into the algorithms, and likely patient responses to different drug therapies can be predicted with high accuracy,” McDonald stated.
Before this research, machine learning efforts often involved a single algorithmic approach. However, McDonald and his team decided to use several algorithm approaches that use multiple methods of analyzing data. The researchers discovered that using this ensemble-based approach significantly boosted the predictive accuracy.
However, more patient datasets are required to advance the organization’s research. McDonald is currently speaking with medical insurance companies about access to relevant datasets. With is information, the researchers can develop methods to decrease medical costs, save time, and improve patient outcomes.
“Right now, a percentage of patients will not respond to a drug, but we don’t know that until after six weeks of chemotherapy,” said McDonald. “What we hope is that we will soon have tools that can accurately predict the probability of a patient responding to first line therapies — and if they don’t respond, to be able to make accurate predictions as to the next drug to be tried.”