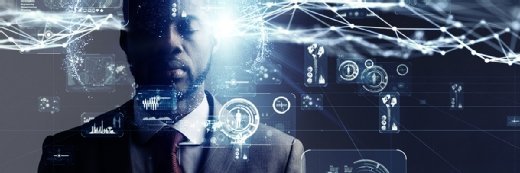
Getty Images
Machine Learning, Predictive Analytics Determine Drug Response
Using machine learning and predictive analytics, researchers can identify cancer patients who will benefit from immunotherapy drugs.
Cleveland Clinic researchers developed a new machine learning model that uses predictive analytics to determine whether immune checkpoint blockade (ICB), a growing class of immunotherapy drugs, will be effective in patients with cancer.
The tool analyzed multiple patient-specific biological and clinical factors to predict the patient’s response to immune checkpoint inhibitors as well as their survival outcome. According to researchers, the tool could help oncologists better identify patients likely to benefit from ICB.
Additionally, patients who would not benefit from ICB could reduce unnecessary expenses and potential side effects. The predictive analytics method could also indicate the need to pursue alternative treatments or a combination.
“It’s important to know which treatment modalities patients are most suited for,” director of Cleveland Clinic’s Center for Immunotherapy & Precision Immuno-Oncology Timothy Chan, MD, PhD, said in a press release.
“Our model provides a more comprehensive understanding of the diversity of responses among patients to immune checkpoint blockade. It’s the first to assemble such a large-scale set of clinical and genomic variables that have predictive value for immunotherapy across numerous cancer types.”
This recent study builds on earlier research by Chan, who found that patients with high tumor mutation burden and DNA repair deficiencies responded well to immune checkpoint therapy.
Immune checkpoints are proteins on specific immune cells that prevent immune responses from damaging or destroying healthy cells when activated. However, some cancer cells can hijack checkpoint signaling and disguise themselves to avoid being targeted by a patient’s immune system. Checkpoint inhibitors can prevent cancer cells from activating the checkpoints.
“However, ICB is not effective in all cancer types. Even in cancers responsive to ICB, half or more of all patients treated with ICB do not derive clinical benefit. Previous research has identified some biomarkers and genomic features associated with ICB efficacy, but no single factor can be considered an optimal predictor of treatment outcomes,” the press release stated.
For the study, Chan and the researcher team created their machine learning model using a dataset containing clinical, tumor, and genetic sequencing information from almost 1,500 patients with 16 different types of cancer. The participants were also treated with two different kinds of immune checkpoint inhibitors or a combination of both.
The researchers then used an algorithm that incorporated many genetic, molecular, clinical, and demographic variables, some of which have been linked to ICB response. The team discovered that the variable with the greatest influence on ICB response was tumor mutational burden, followed by the patient’s chemotherapy history.
The hemoglobin, platelets, and albumin levels also indicated strong predictive value for patient survival rates and treatment response.
“How these variables all work together is the key here,” said Chan. “This model shows that, rather than a single predictive biomarker, we’re headed toward a multifactor nomogram for clinical use.”
The team’s predictive model proved to be highly accurate and outperformed two other forecasting tools.
“The model works well, despite what type of cancer is being assessed, which shows that these commonalities are what’s important,” Chan explained. “These are primary factors that affect ICB response. The factors may be weighted a little bit differently from cancer to cancer, but it’s almost like a common language for response prediction.”
According to researchers, the positive results support their motion to move forward with testing the model in a clinical trial with a diverse population of cancer patients.