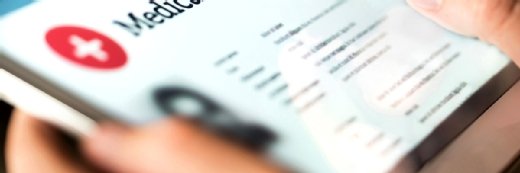
terovesalainen - stock.adobe.com
Predictive Model Determines Antidepressant Medication Response
A noninvasive predictive model can determine how a patient will respond to certain antidepressant medications, preventing unnecessary side effects.
Using data from the National Institute of Mental Health, UT Southwestern Medical Center researchers created a predictive model to determine how a patient will respond to antidepressants.
According to researchers, these findings could provide a strong precision medicine approach in identifying the correct medication for individuals.
“This is a significant advance. It’s noninvasive. It can be and should be used immediately,” Professor of Clinical Psychiatry and Director of the Center for Depression Research and Clinical Care, Madhukar Trivedi, MD, said in a press release.
Trivedi explained that the new biomarkers could prevent patients from taking the wrong medication and dealing with unnecessary side effects. The study tested the common antidepressant drug sertraline with a control group taking a placebo. Those that did not respond to sertraline after eight weeks switched to the antidepressant bupropion.
Researchers then measured the changes in brain circuit reactions while the patients performed a reward task in the scanner. Noninvasive functional magnetic resonance imaging (fMRI) was conducted in more than 300 patients to examine changes in brain function at rest and during the reward task.
Using the data, the researchers constructed machine learning models to tell medical professionals which specific brain regions and circuits were linked to the prediction of the treatment response.
“The signatures that we found are unique to each antidepressant’s response,” said Albert Montillo, PhD, Assistant Professor of the Lyda Hill Department of Bioinformatics, whose lab developed the 10,000 lines of code to efficiently train the new predictive models to achieve extreme levels of accuracy.
“Due to the human brain’s inherent complexity, neuroscientists typically find that brain activity can explain 15 percent of the variance in symptom relief. That would be a significant scientific finding. Twenty percent is huge,” Montillo continued. “In this study, we’re able to explain 48 percent of the variance in the symptom relief from sertraline, 34 percent for bupropion, and 28 percent for placebo.”
According to researchers, the study is one of the first adaptations of deep machine learning to predict antidepressant treatment outcomes.
“It is a clear improvement from the standard prediction approaches currently used,” Dr. Trivedi said. “We have also reached a point where our findings are stable and can provide a pathway for future work.”
Montillo added, “The analytical approach we have developed can be readily adapted to identify biomarker signatures and predict outcomes for other treatments of depression, both pharmacological and non-pharmacological.”
The research team plans to seek additional funding to advance their research and see if the prediction model is compatible with blood biomarkers.