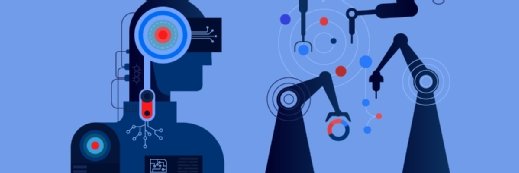
Getty Images
Machine Learning Tool Predicts Kidney Function Response To Treatment
Researchers created a machine learning tool to predict if certain drugs are effective treatment options to protect kidney function for patients with lupus nephritis.
Using predictive analytics, researchers developed a machine learning tool to determine how lupus nephritis patients will respond to specific treatment options.
Inflammatory kidney disease occurs in about half of patients with systemic lupus erythematosus (SLM). Additionally, nearly half of lupus nephritis patients will develop chronic and potentially end-stage kidney disease that requires dialysis or kidney transplant.
“Many factors contribute to lupus nephritis, but most patients have lesions with inflammatory cells that invade the capillaries in the kidney, blocking these vessels and leading to kidney dysfunction. This damage can cause irreversible scarring and fibrosis, which in turn may lead to other health problems, such as heart attacks, strokes, infections, and even death,” the press release stated.
Currently, the standard treatment for an individual diagnosed with lupus nephritis is one of two immunosuppressive drugs given as a trial for six months. After six months, physicians can assess how the patient is responding to treatment.
If there is not a positive response, the FDA approved several second-line drugs to be used instead. However, those drugs are expensive and are usually reserved for known nonresponders.
Clinicians face the challenge of deciding when to add second-line drugs and other resources for patients who are not responsive to the initial treatment. According to researchers, irreversible kidney damage can occur during the trial period.
To assist physicians, Jim Oates, a professor of medicine and Director of the Division of Rheumatology and Immunology at the Medical University of South Carolina, and his colleagues created a novel prediction tool.
“We want to find the patients who are most at risk for poor outcomes but whose outcomes can be reversed,” Oates said.
The researchers developed a predictive analytics tool by examining different variables and disease indicators to determine how a patient’s disease will respond to treatment.
According to the press release, “In a rheumatology clinic where physicians see lupus patients day in and day out, the specialists look at the whole picture to decide on treatment and how to monitor patients. The tool mirrors that holistic perspective and may provide a useful clinical aid for clinicians who do not encounter SLE and lupus nephritis as often.”
The prediction tool uses machine learning to assist healthcare providers in submitting specific pathology and laboratory values. The tool can then determine the likelihood that a patient will respond to therapy within one year.
After testing the tool on a population representative of the clinic, the team narrowed the testing results to seven key indicators they used to develop the tool.
These are the International Society of Nephrology/Renal Pathology Society biopsy scores for activity, chronicity, interstitial fibrosis, and interstitial inflammation as well as the urine protein-to-creatinine ratio, white blood cell count, and hemoglobin level. Based on the values, the tool uses predictive analytics to determine if a patient will respond to treatment.
According to researchers, the tool can help physicians identify which patients will benefit from extra assistance or more frequent monitoring.
“The ultimate goal for helping lupus nephritis patients is to preserve as much kidney function as possible. Knowing that a patient is not likely to respond to the initial therapy may mean lowering the threshold for when to change therapy, perhaps not waiting the full 6 months of a standard trial,” the press release stated.
The research team plans to expand and validate the tool with different populations and other institutions. Additionally, the lab is working on other prediction models. However, some of the biomarkers in those studies are not widely available or require further research on genetic markers.
Oates is grateful that even in the early stages of the tool’s development, it can start delivering immediate benefits.
“I delved into making this tool because the variables are available and can make an impact now,” Oates said. “And I’ve been amazed by the high percentage of lupus patients who agreed to be part of this long-term study to help their fellow patients. They make this work possible.”