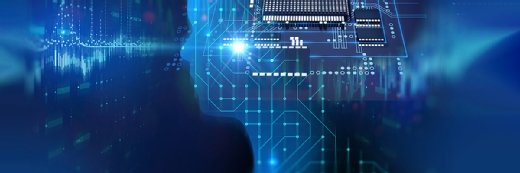
Getty Images
Machine Learning Gauges Unconsciousness Under Anesthesia
A machine learning tool can accurately assess unconsciousness in patients under anesthesia.
Machine learning can measure unconsciousness in patients under anesthesia, allowing anesthesiologists to optimize drug doses, according to a study published in PLOS One.
Anesthetic drugs act on the brain but most anesthesiologists rely on heart rate, respiratory rate, and movement to infer whether surgery patients remain unconscious to the desired degree.
A team from MIT and Massachusetts General Hospital (MGH) showed that a machine learning approach, attuned to the kind of anesthetic being used, can yield algorithms that evaluate unconsciousness in patients based on brain activity with high accuracy and reliability.
"One of the things that is foremost in the minds of anesthesiologists is 'Do I have somebody who is lying in front of me who may be conscious and I don't realize it?'" said senior author Emery N. Brown, Edward Hood Taplin Professor in The Picower Institute for Learning and Memory and the Institute for Medical Engineering and Science at MIT, and an anesthesiologist at MGH.
“Being able to reliably maintain unconsciousness in a patient during surgery is fundamental to what we do. This is an important step forward."
In addition to providing an accurate assessment of unconsciousness, the new algorithms offer anesthesiologists the potential to maintain unconsciousness at the desired level while using less drug than they might administer otherwise when depending on less accurate and reliable indicators.
This could improve patients’ post-operative outcomes like delirium. For example, when used to drive an infusion pump, algorithms could help anesthesiologists precisely measure drug delivery to optimize a patient’s state and the doses they receive.
"We may always have to be a little bit 'overboard'," said Brown, who is also a professor at Harvard Medical School. "But can we do it with sufficient accuracy so that we are not dosing people more than is needed?"
For the study, researchers trained machine learning algorithms on a dataset collected in 2013. In that project, ten healthy volunteers underwent anesthesia with a commonly used drug, propofol. As the dose was methodically raised using computer-controlled delivery, researchers asked volunteers to respond to a simple request until they couldn’t anymore.
When they were brought back to consciousness as the dose was later lessened, they were able to respond again. As the doses were increasing and decreasing, the team recorded neural rhythms reflecting their brain activity using electroencephalogram (EEG) electrodes. This provided a direct, real-time link between measured brain activity and exhibited unconsciousness.
In the new study, the team trained versions of their AI algorithms on more than 33,000 two-second-long snippets of EEG recordings from seven of the volunteers. This way, the algorithms could learn the difference between EEG readings predictive of consciousness and unconsciousness under anesthesia.
The group then used the algorithms to analyze EEG recorded from 27 real surgery patients who received propofol for general anesthesia. The algorithms were able to distinguish unconsciousness with a higher level of accuracy than other studies have shown.
In one case, the algorithms were able to detect a patient’s decreasing level of unconsciousness several minutes before the actual attending anesthesiologist did. This shows that if it had been in use during the surgery itself, it could have provided an accurate and helpful early warning.
The team also applied the algorithms to EEG recordings from 17 surgery patients who were anesthetized with sevoflurane. Sevoflurane is different from propofol and is inhaled instead of infused, but it works in a similar way.
Researchers found that the algorithms performed with high, but somewhat reduced accuracy, indicating that their ability to classify unconsciousness carried over reliably to another anesthetic drug that works in a similar way.
The ability to predict unconsciousness across different drugs with the same mechanism of action is critical. Current EEG-based systems for monitoring consciousness don’t distinguish among drug classes, even though different categories of anesthesia drugs work in different ways. These systems also don’t adequately account for known age differences in brain response and anesthesia.
Researchers noted that they want to train the machine learning system distinctly for use with children or seniors. The team can also train new algorithms to apply specifically for other kinds of drugs with different mechanisms of action.
The system is also very easy to implement and extend to other organizations, researchers stated.
“This is a proof of concept showing that now we can go and say let’s look at an older population or let’s look at a different kind of drug,” said John Abel, a postdoc and leader of the study. “Doing this is simple if you set it up the right way.”
The team is working to refine the algorithms, and to expand testing to hundreds more cases to confirm their performance.