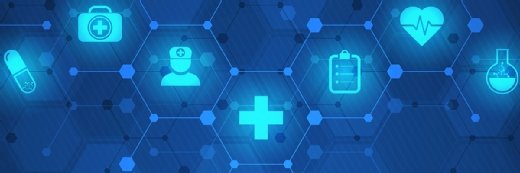
bestbrk/istock via Getty Images
Artificial Intelligence Can Help Robots Navigate the ED
The artificial intelligence algorithm can take into account how many people are gathered together in the ED and how quickly these people are moving.
Researchers at the University of California San Diego have developed an artificial intelligence algorithm that can help robots better navigate the ED.
The team has also developed a dataset of open-source videos to help train robotic navigation systems in the future.
Robots would best help clinicians, nurses, and staff in the emergency department by delivering supplies and materials, but this means robots have to know how to avoid situations where clinicians are busy caring for a patient in serious or critical condition.
“To perform these tasks, robots must understand the context of complex hospital environments and the people working around them,” said Laurel Riek, a professor of computer science and emergency medicine at UC San Diego.
Researchers built a navigation system, called the Safety Critical Deep Q-Network (SafeDQN), around an artificial intelligence algorithm that takes into account how many people are clustered in a space and how quickly these people are moving.
This is based on how clinicians behave in the emergency department, the group noted: When a patient’s condition worsens, a team immediately gathers around them to deliver care. Clinicians’ movements are quick, alert, and precise, and the navigation system directs the robots to move around these clustered groups of people, staying out of the way.
“Our system was designed to deal with the worst-case scenarios that can happen in the ED,” said Angelique Taylor, a PhD student who is part of Riek’s Healthcare Robotics lab at the UC San Diego Department of Computer Science and Engineering.
Researchers trained the algorithm on videos from YouTube, mostly from documentaries and reality shows. The collection of more than 700 videos is available for other research teams to train other algorithms and robots.
The team tested the AI algorithm in a simulation setting and compared its performance to other state-of-the-art robotic navigation systems. The results showed that the SafeDQN system generated the most efficient and safest paths in all cases.
Going forward, researchers will test the system on a physical robot in a realistic environment. The team plans to partner with UC San Diego Health researchers who operate the campus’s healthcare training and simulation center. The group also noted that the algorithms could be used outside of the ED.
Researchers have increasingly leveraged artificial intelligence and other data analytics tools to improve standard care processes.
A team from MIT and Massachusetts General Hospital (MGH) recently showed that machine learning can measure unconsciousness in patients under anesthesia, allowing anesthesiologists to optimize drug doses.
“One of the things that is foremost in the minds of anesthesiologists is 'Do I have somebody who is lying in front of me who may be conscious and I don't realize it?'” said senior author Emery N. Brown, Edward Hood Taplin Professor in The Picower Institute for Learning and Memory and the Institute for Medical Engineering and Science at MIT, and an anesthesiologist at MGH.
“Being able to reliably maintain unconsciousness in a patient during surgery is fundamental to what we do. This is an important step forward.”
MIT and MGH researchers want to train the machine learning system distinctly for use with seniors or children. The group also wants to train new algorithms to apply specifically for other kinds of drugs with different mechanisms of action.
“This is a proof of concept showing that now we can go and say let’s look at an older population or let’s look at a different kind of drug,” said John Abel, a postdoc and leader of the study. “Doing this is simple if you set it up the right way.”