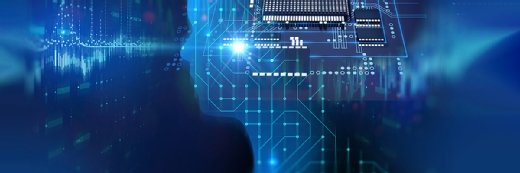
Getty Images
Deep Learning Analyzes CT Scans to Determine Cancer Type
The deep learning network could help radiologists assess lung cancer types on CT scans, leading to more personalized treatment plans.
Using deep learning, radiologists could more accurately evaluate lung cancer types on CT scans and improve treatment plans, according to a study published in the Journal of Medical Imaging.
The correlation of molecular patterns, like gene expression and mutation, with image features is commonly referred to as radiogenomics, researchers noted. This field is limited by its frequent use of high-dimensional data, where the number of features exceeds that of observations.
The field of radiogenomics is also hindered by several simplifying model assumptions and a lack of validation datasets. While deep learning techniques can alleviate this situation by providing accurate predictions of image features from gene expression patterns, researchers often don’t know what the model has learned.
"The ability to interrogate the model is critical to understanding and validating the learned radiogenomic associations," said William Hsu, associate professor of radiological sciences at the University of California, Los Angeles, and director of the Integrated Diagnostics Shared Resource.
Using a dataset of 262 patients, the team trained the deep learning networks to predict 101 features from a collection of 21,766 gene expressions. Researchers then tested its predictive ability on an independent dataset of 89 patients, while comparing its ability against that of other models within the training dataset.
Finally, researchers applied gene masking to determine the learned associations between subsets of genes and the type of lung cancer.
The results showed that the deep learning networks were able to represent these datasets better than the other models and were generalizable to datasets from another population.
Additionally, the results of gene masking indicated that the prediction of each imaging feature was related to a unique gene expression profile governed by biological processes.
"While radiogenomic associations have previously been shown to accurately risk stratify patients, we are excited by the prospect that our model can better identify and understand the significance of these associations,” said Hsu.
“We hope this approach increases the radiologist's confidence in assessing the type of lung cancer seen on a CT scan. This information would be highly beneficial in informing individualized treatment planning.”
Researchers have previously applied deep learning approaches to lung cancer treatment planning. A study published in the International Journal of Medical Informatics showed that a deep learning tool was able to accurately predict survival expectancy in patients with lung cancer, potentially leading to more informed care decisions by providers.
The tool can analyze large amounts of data that describe the patients and the disease to understand how a combination of factors affect lung cancer survival periods. These factors include information like types of cancer, size of tumors, speed of tumor growth, and demographic data.
“This is a high-performance system that is highly accurate and is aimed at helping doctors make these important decisions about providing care to their patients,” said Youakim Badr, associate professor of data analytics at Penn State Great Valley. “Of course, this tool can’t be used as a substitute for a doctor in making decisions on lung cancer treatments.”
The structure of deep learning offers several advantages for many data science tasks, especially in cases involving large datasets.
“It improves performance tremendously. In deep learning we can go deeper, which is why they call it that. In traditional machine learning, you have a simple structure of layers of neural networks. In each layer, you have a group of cells,” said Robin G. Qiu, professor of information science and engineering and an affiliate of the Institute for Computational and Data Sciences.
“In deep learning, there are many layers of these cells that can be architected into a sophisticated structure to perform better feature transformation and extraction, which gives you the ability to further improve the accuracy of any model.”