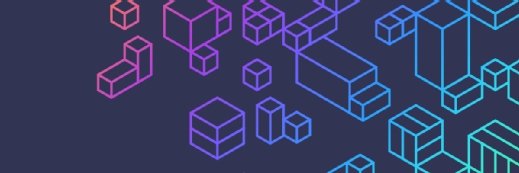
Limiting Bias in Artificial Intelligence Tools, Personalized Medicine
Researchers are developing data collection methods to decrease bias in artificial intelligence tools and precision medicine.
As more research looks to use medical data for artificial intelligence tools and personalized medicine, a new study shows how unintentional bias can impact design efforts.
Additionally, the study highlights methods to increase the likelihood of patients from underrepresented populations being included in massive banks of genetic samples and data from electronic medical records. According to researchers, diverse data benefits both the accuracy of tools and provides better patient outcomes for underrepresented communities.
The findings come from researchers at the University of Michigan and Michigan State University that studied the University of Michigan’s efforts to build a large bank of data and samples for researchers to use.
According to researchers, the study results have already improved how Precision Health at U-M recruits participants and the racial and ethnic categories that patients can self-select to add to their records.
The study focuses on the Michigan Genomics Initiative (MGI), which designed its recruitment efforts around approaching individuals to donate small blood samples for the research biobank while awaiting surgery at Michigan Medical. The trained MGI recruiters aimed to approach all adult surgical patients in the preoperative setting during surgical hours.
“There were several reasons why MGI used this approach — including the fact that patients in such settings have time to engage in recruitment and enrollment procedures, and that they often already have an intravenous line placed in preparation for their treatment, so it’s convenient to draw a blood sample for research use if they consent,” the press release stated.
However, researchers found that the pool of surgical patients from which MGI staffer recruited was predominantly older, White, and socioeconomically advanced men compared to the general Michigan Medicine patient population.
Additionally, when approached, patients who consented to enroll were younger than the average patient waiting for surgery and were less likely to be Black, Asian, or Hispanic. The lack of diverse data collection resulted in blood samples for biobank representing only a sub-population that was less demographically diverse than the overall patient population.
To expand their outreach efforts, Precision Health now includes a mail-in-saliva-collection kit, increasing engagement with a broader patient population.
“We know that large research datasets often do not reflect the diversity of the patient population across the United States, but our study gives a detailed analysis about how these disparities become embedded in scientific advances from the ground up,” said Kayte Spector-Bagdady, JD, MBE, co-first author of the new paper and a research ethicist at Michigan Medicine.
“This way we were able to highlight practical improvements that we could implement immediately.”
The study was led by Spector-Bagdady, a U-M Medical School assistant professor who is the Associate Director of U-M’s Center for Bioethics and Social Sciences in Medicine and senior author Jenna Wiens, PhD, one of the co-directors of Precision Health and an associate professor of computer science and engineering at the U-M College of Engineering.
“A lot of the research that goes on in precision health, machine learning, and AI for health care across the country leverages data from the electronic health records of major health systems, and data from the subset of patients who have consented to give biospecimens,” Wiens explained.
“For an AI researcher who builds machine learning and clinical decision support tools, generalizability is so important. Otherwise, we risk building tools that perpetuate disparities in care and outcomes.”
The authors note that many academic medical centers, including Michigan Medicine, inform patients when they consent to receive care that their medical records might be used for research. Taking part in MGI involves allowing those records to be used in conjunction with a sample of their DNA.
“For instance, researchers might analyze part of their genetic sequence and look at how their genetic traits relate to conditions they have or how well they do when given certain treatments. This is a powerful tool for understanding what drives certain diseases, or what treatments work best for people with different characteristics who have the same type of cancer, for instance,” the press release stated.
Additionally, data can also help create AI tools to predict patient complications or select the best treatment options. By collecting diverse data, researchers can develop better precision medicine and AI strategies to improve patient outcomes.