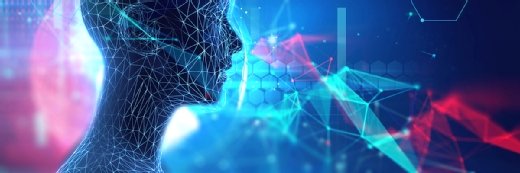
Getty Images
Predictive Model Detects Early-Stage Lung Cancer
A predictive model can detect lung cancer when patients are asymptomatic by analyzing blood samples.
According to a study by Massachusetts General Hospital (MGH) researchers, a predictive model can detect early-stage lung cancer from alterations in blood metabolites.
Lung cancer is the leading cause of cancer death and is typically diagnosed at a late stage when the survival rate is extremely low. Early-stage lung cancer is mostly asymptomatic, and low-dose spiral CT imaging is not
a viable option to conduct widespread screenings for the general population.
The MGH study provides proof of concept that a drop of blood can reveal lung cancer in asymptomatic patients.
“Our study demonstrates the potential for developing a sensitive screening tool for the early detection of lung cancer,” associate biophysicist, Leo Cheng, PhD, said in a press release.
“The predictive model we constructed can identify which people may be harboring lung cancer. Individuals with suspicious findings would then be referred for further evaluation by imaging tests, such as low-dose CT, for a definitive diagnosis.”
The research team built a lung cancer predictive model based on metabolic profiles in blood. Metabolomic analyzes cellular metabolite flows to decipher healthy and pathological states by examining the metabolome.
Lung cancer can cause changes in the blood metabolites produced or consumed by cancer cells in the lungs. The research team measured metabolomics profiles in blood using high-resolution magnetic resonance spectroscopy. This tool can analyze an array of compounds within living cells by measuring the collective reactions of metabolites.
The researchers examined tens of thousands of blood specimens stored in MGH’s biobank and others. The team found 25 patients with non-small cell lung cancer (NSCLC) with stored blood specimens obtained at the time of their diagnosis and at least six months before their diagnosis. They matched these patients with 25 healthy controls.
Researchers first trained the predictive model to recognize lung cancer by measuring metabolomic profile values in blood samples from patients at the time of their diagnosis and comparing them to blood samples from the healthy controls.
The model was then validated using blood samples from the same patients obtained before their lung cancer diagnosis. The predictive model yielded values between the healthy controls and the patients at the time of their diagnosis.
“This was very encouraging, because screening for early disease should detect changes in blood metabolomic profiles that are intermediate between healthy and disease states,” said Cheng.
The research team tested their model with a different group of 54 patients with NSCLC using blood samples obtained before their cancer diagnosis, confirming that the model’s predictions were accurate. Additionally, values from the predictive model could predict five-year survival for patients, which could aid in guiding clinical strategies and treatment decisions.
Next, the research team will study metabolomic profiles of lung cancer’s clinical characteristics to understand the entire metabolic spectrum of the disease, which could lead to precision medicine and targeted therapies. The team also plans to use the same technology to screen for Alzheimer’s disease using blood samples and cerebrospinal fluid.
With the predictive model, researchers can develop strategies for early intervention and preventive care.